PaperLedge
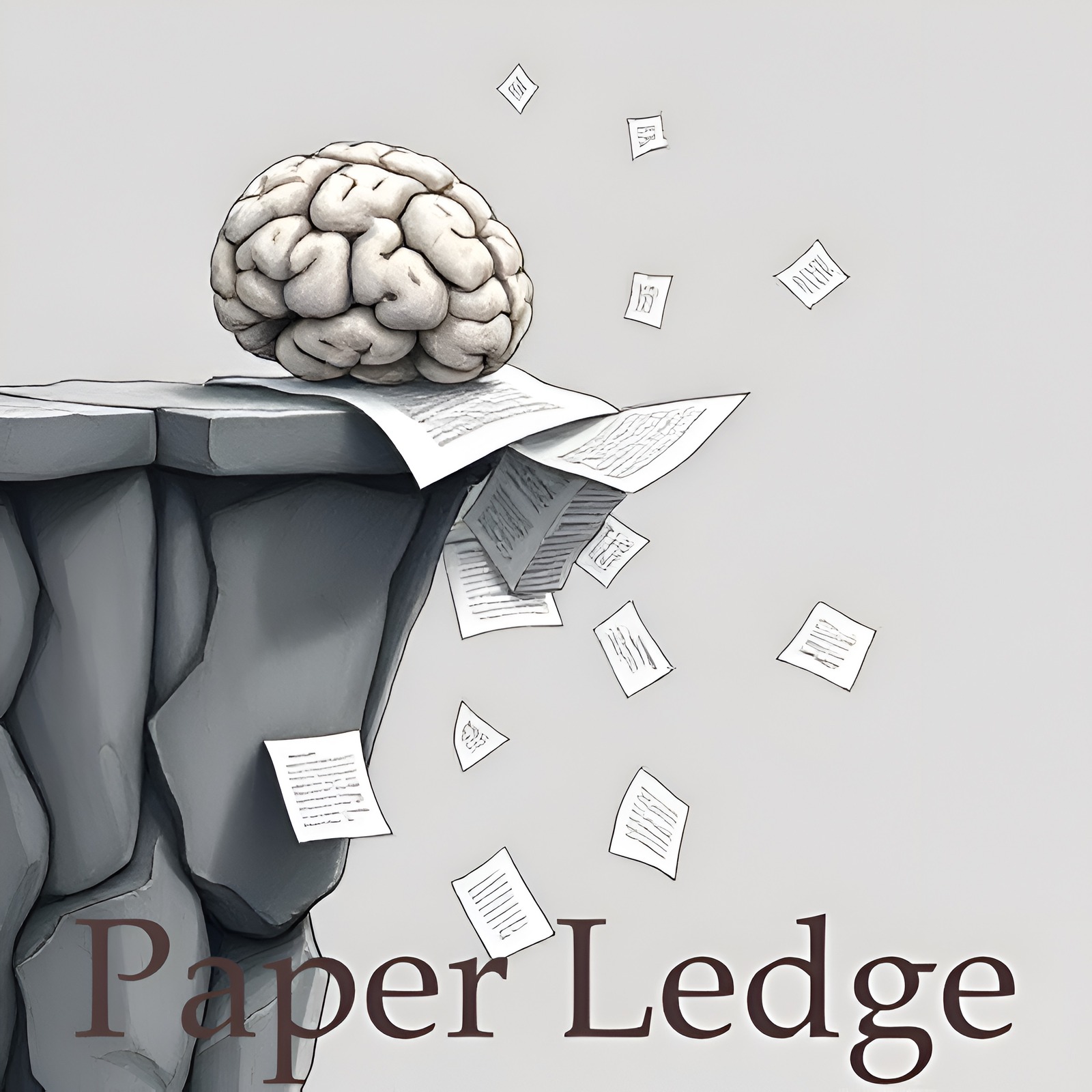
PaperLedge where research meets storytelling is a revolutionary podcast where cutting-edge research meets AI-powered storytelling. Hosted by the Ernis, whose blend of gentle reassurance, cosmic wonder, explanatory clarity, and enthusiastic charm makes complex research accessible to everyone. Each episode, Ernis transforms the latest academic papers into engaging, jargon-free audio experiences that deliver key insights in digestible formats. Whether you’re a researcher seeking interdisciplinary perspectives, a student supplementing your studies, or simply curious about scientific breakthroughs, PaperLedge has something for you.
Episodes
Episodes
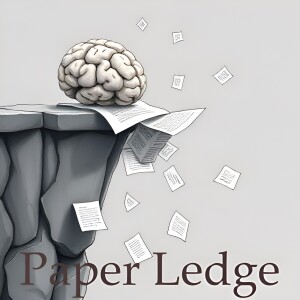
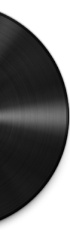
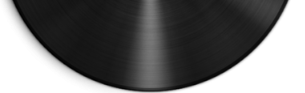
37 minutes ago
37 minutes ago
Alright, Learning Crew, Ernis here, ready to dive into another fascinating paper! This time, we're tackling something that every software developer knows and dreads: merge conflicts.
Imagine you're working on a group project, like building a house. You and your friends are all making changes – one's painting the walls, another's installing the plumbing. Now, what happens if you both try to change the same wall at the same time? That's a conflict! In software, that's when two developers change the same part of the code in different versions, or branches, and then try to combine them.
These conflicts can be simple, like two people editing the same sentence (textual conflicts), or much more complicated, like one person removing a crucial part that the other person's new code relies on (build and test conflicts). Either way, they slow everything down and can lead to buggy software. No fun!
Now, there are tools out there to help find these conflicts, but this paper focuses on resolving them, especially the tricky ones. Existing tools often struggle when, say, a developer removes a whole method – a mini-program within the larger program. That's where BUCOR comes in. Think of BUCOR as a super-smart mediator for code conflicts.
BUCOR works by comparing three versions of the code: the original, the version with your changes, and the version with your teammate's changes. It then uses a two-pronged approach:
Rule-Based Transformation (BUCOR-R): This is like having a set of pre-written recipes for common conflicts. If it sees a conflict it recognizes, it applies the fix automatically. Think of it like knowing that if someone spills coffee on the floor, you grab a mop – a standard solution for a standard problem.
Example-Based Transformation (BUCOR-E): This is where things get really clever. BUCOR looks at other times similar conflicts have been fixed in the project's history. It learns from those examples and figures out how to apply a similar solution to the current problem. It's like learning from watching a master chef – you pick up techniques and adapt them to new ingredients.
So, BUCOR combines a "rule book" with a "learning brain" to tackle merge conflicts.
The researchers tested BUCOR on 88 real-world conflicts and found that it could come up with a solution for most of them. Even better, it correctly resolved almost half of the conflicts entirely on its own! This shows that this "hybrid" approach – combining rules and learning – is really promising for making merge conflict resolution much easier.
"Our research sheds light on future directions for more intelligent and automated merge tools."
So, why does this matter to you? Well:
For Developers: This could mean less time wrestling with merge conflicts and more time building awesome software!
For Project Managers: This could lead to faster development cycles and higher quality code.
For End Users: This could result in fewer bugs and a better overall software experience.
This research highlights the potential for smarter, more automated tools to help developers collaborate more effectively. But it also raises some interesting questions:
How much human oversight is still needed when using a tool like BUCOR? Can we ever truly trust a machine to resolve complex code conflicts on its own?
Could this approach be applied to other types of conflicts, like conflicts in documents or databases?
As AI gets even better, what are the ethical implications of letting machines make decisions about code changes?
Lots to think about, Learning Crew! This paper opens the door to a future where merge conflicts are less of a headache and more of a solved problem. I'm excited to see where this research leads!Credit to Paper authors: Sheikh Shadab Towqir, Fei He, Todd Mytkowicz, Na Meng
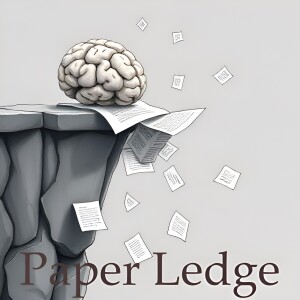
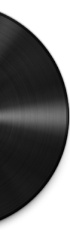
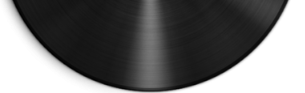
40 minutes ago
40 minutes ago
Hey PaperLedge crew, Ernis here, ready to dive into some fascinating research! Today, we’re exploring how we can make smart homes even smarter, especially for those who might find today’s tech a bit tricky – like our older adults or folks with impairments.
Think about it: a smart home is supposed to make life easier, right? But sometimes, the touchscreens, voice commands, and apps can be frustrating. So, some clever researchers over at the HASE research group, part of the Living Lab Kobo, are asking: "What if we could control our homes with just a thought or a blink?"
That’s the core of this paper. They've been tinkering with something called _Sagacity_, a comprehensive smart home management system. And they're exploring how bioelectric signals – specifically EMG and EOG – could act as a complementary interface.
EMG? That's electromyography, which basically reads the electrical activity of your muscles. Imagine twitching your cheek to turn on the lights!
EOG is electrooculography, which tracks your eye movements. So, maybe a certain blink pattern could adjust the thermostat.
Now, before you think this is straight out of a sci-fi movie, remember it’s preliminary research. But the potential is huge!
What makes this study especially cool is their approach. They didn’t just sit in a lab. They ran interactive workshops with older adults and impaired persons – 18 subjects total – getting their hands-on feedback. It’s all about participatory research, ensuring the tech actually meets the needs of the people who'll be using it.
“We investigated the potential of bioelectric signals, in particular EMG and EOG as a complementary interface for SHT… The preliminary insights from the study unveil the potential of EMG/EOG interfaces in multimodal SHT management…”
Think of it like this: imagine trying to design a new type of shoe without ever talking to people who wear shoes. You might end up with something that looks cool but is totally impractical! This study prioritizes user input, making the research relevant.
So, what did they find? Well, the initial results are promising! They see the potential of using EMG and EOG alongside existing interfaces like voice control. It's not about replacing everything, but about adding another layer of accessibility.
However, they also identified some challenges. The technology isn't perfect yet, and there are limitations to overcome. The research also provides great recommendations for designing multimodal interaction paradigms pinpointing areas of interest to pursue in further studies
For example, current EMG/EOG sensors can be a bit clunky. And figuring out the right eye movements or muscle twitches to trigger specific actions will take time and lots of user feedback.
So, why does this matter? Well, for our older listeners or those with impairments, this research offers a glimpse of a future where technology truly adapts to them, rather than the other way around. For designers and engineers, it’s a call to think beyond standard interfaces and embrace innovative, inclusive solutions. And for all of us, it’s a reminder that technology should be about empowerment and accessibility for everyone.
This study is not just about tech, it's about inclusivity and improving the lives of those who might be left behind by the rapid pace of technological advancement.
Now, a couple of things that popped into my head while reading this:
How do we ensure these bioelectric interfaces are secure and private? Could someone potentially "hack" your eye movements to control your home?
And, what are the ethical considerations of using technology that directly interfaces with our bodies? Where do we draw the line?
Definitely some food for thought, crew! Let me know what you think. Until next time, keep those neurons firing!Credit to Paper authors: Wiesław Kopeć, Jarosław Kowalski, Aleksander Majda, Anna Duszyk-Bogorodzka, Anna Jaskulska, Cezary Biele
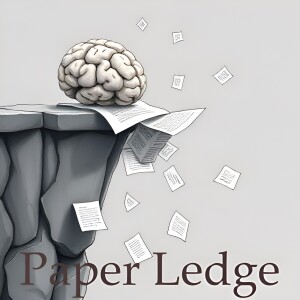
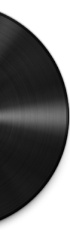
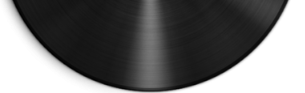
5 days ago
5 days ago
Hey PaperLedge crew, Ernis here, ready to dive into some fascinating research! Today, we're tackling a paper that's all about making some pretty cool tech more accessible to everyone. You know how some cameras can see things that regular cameras can't, like how light is polarized? That special ability can help us see hidden details, enhance images, and even create 3D models. Think of it like having super-powered vision!
But here's the catch: these "polarization cameras" aren't exactly common. They can be expensive and tricky to use, which limits who can really play around with this tech. That's where this research comes in. The goal? To create realistic polarization images using just a regular, everyday photo. It's like turning a standard picture into a super-vision image, all with the help of some clever algorithms.
Now, in the past, scientists have tried to simulate polarization using computer programs, but these programs needed a ton of information. They needed detailed 3D models of objects and their materials, which takes a lot of time and effort to create. It’s like trying to build a virtual world from scratch just to test out this polarization trick. The paper introduces PolarAnything, a new method that changes the game.
So, what's so special about PolarAnything? Well, it's based on something called a "diffusion model." Think of it like this: imagine you have a blurry image, and you slowly add noise to it until it's completely unrecognizable. A diffusion model learns how to reverse that process – how to take that noisy mess and gradually turn it back into a clear, detailed image. In this case, the research team trained a diffusion model to generate polarization information based on a regular photo. Pretty neat, huh?
No more reliance on complex 3D models: PolarAnything works directly from a single RGB image.
Photorealistic Results: The generated polarization images look incredibly real.
Physically Accurate: The polarization properties are not just visually appealing, but also scientifically sound.
The real magic lies in how they represent this polarization information. It's like finding the right code to unlock hidden details in the image. And the best part? This model is remarkably effective. It can generate high-quality polarization images that are not only visually convincing but also physically accurate. This means they can be used for other cool applications, like "shape from polarization," which is basically figuring out the 3D shape of an object just by looking at how light is polarized on its surface.
This is important because it opens up a whole new world of possibilities. Imagine:
Better medical imaging: Seeing subtle tissue differences that are normally invisible.
Improved object recognition in self-driving cars: Helping cars "see" better in challenging lighting conditions.
More realistic augmented reality: Creating AR experiences that seamlessly blend virtual objects with the real world.
"PolarAnything eliminates the dependency on 3D asset collections."
So, what does all this mean for you, the PaperLedge listener? Well, if you're a researcher, this could give you a powerful new tool for your work. If you're a tech enthusiast, it's a glimpse into the future of image processing. And if you're just curious about the world around you, it's a reminder that there's always more to see than meets the eye.
Now, some questions that popped into my head while reading this paper:
How well does PolarAnything handle really complex scenes with lots of different materials and lighting conditions?
Could this technology be adapted to work with video, creating real-time polarization effects?
That's all for this episode, PaperLedge crew! Until next time, keep exploring and keep learning!Credit to Paper authors: Kailong Zhang, Youwei Lyu, Heng Guo, Si Li, Zhanyu Ma, Boxin Shi
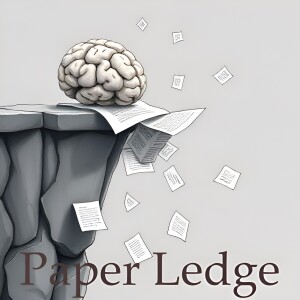
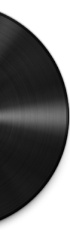
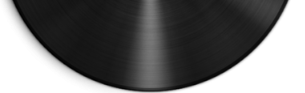
5 days ago
5 days ago
Hey PaperLedge crew, Ernis here, ready to dive into some cutting-edge AI that could revolutionize how we understand and diagnose diseases! Today, we're looking at a paper about using really smart computer programs, called multimodal large language models (MLLMs), to analyze pathology images – think of slides under a microscope.
Now, pathology is where doctors examine tissue samples to figure out what's going on inside your body. Traditionally, this is done by highly trained pathologists, but it can be time-consuming and requires a lot of expertise. What if we could teach a computer to help?
That's where MLLMs come in. Imagine you're trying to understand a complex scene. You don't just look at it; you also use language to describe it, ask questions, and connect it to your existing knowledge. MLLMs do the same thing! They can "see" the pathology image and "understand" written information about it, allowing them to make much more informed judgments.
But here's the catch: previous attempts to use MLLMs in pathology have been a bit… limited. They've struggled with complex reasoning, often relying on expensive and time-consuming human explanations to guide them. And they've mostly focused on small areas of the image, missing the bigger picture. Think of it like trying to understand a novel by only reading individual sentences out of context.
That's where this new research comes in! The paper introduces something called SmartPath-R1, a souped-up MLLM designed to overcome these limitations. It's like giving the AI a pair of super-powered glasses and a textbook all in one!
The key innovation is how they trained SmartPath-R1. Instead of relying on humans to explain every single step of the reasoning process (which is super expensive!), they used a clever technique called task-aware reinforcement fine-tuning. Think of it like teaching a dog a trick. You don't explain every muscle movement; you just reward the dog when it gets closer to the desired behavior. SmartPath-R1 learns by getting "rewards" for making accurate diagnoses.
But wait, there's more! SmartPath-R1 can handle both small regions of interest and entire slides! It uses a mixture-of-experts mechanism, which is like having a team of specialists, each focusing on a different aspect of the image. This allows it to dynamically adapt to different tasks, from identifying specific cells to classifying entire tissue samples.
"This work represents a significant step toward developing versatile, reasoning-enhanced AI systems for precision pathology."
To train and test SmartPath-R1, the researchers put together a massive dataset of 2.3 million region-of-interest samples and 188,000 whole-slide images! That's a lot of data! And the results were impressive. Across 72 different tasks, SmartPath-R1 outperformed existing methods, demonstrating its effectiveness and versatility.
For doctors: Faster and more accurate diagnoses, potentially leading to earlier and more effective treatments.
For researchers: A powerful new tool for understanding disease mechanisms and developing new therapies.
For patients: Peace of mind knowing that your diagnosis is based on the best available technology.
So, what does all this mean? It means we're one step closer to a future where AI can help doctors diagnose diseases more accurately and efficiently, ultimately improving patient outcomes.
Now, a few things to ponder:
How do we ensure that these AI systems are used ethically and responsibly, especially when it comes to patient privacy?
Could AI eventually replace human pathologists, or will it always be a tool to augment their expertise?
How do we build trust in these AI systems, especially when they make decisions that are difficult to understand?
That’s all for today, crew! Keep learning, and keep questioning!Credit to Paper authors: Zhe Xu, Ziyi Liu, Junlin Hou, Jiabo Ma, Cheng Jin, Yihui Wang, Zhixuan Chen, Zhengyu Zhang, Zhengrui Guo, Fengtao Zhou, Yingxue Xu, Xi Wang, Ronald Cheong Kin Chan, Li Liang, Hao Chen
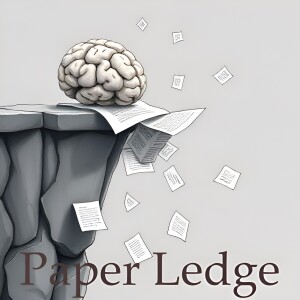
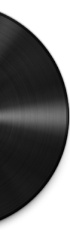
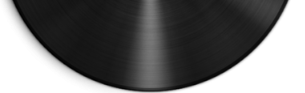
5 days ago
5 days ago
Hey PaperLedge crew, Ernis here, ready to dive into some seriously cool research that could change the way we see our roads! Today we're talking about a new way to spot potholes, cracks, and other road damage, and it's all about combining seeing with reading.
Think about it: a picture is worth a thousand words, right? But what if you also had the thousand words? That's the problem this paper tackles. Existing systems that try to automatically find road damage rely solely on cameras. But a picture alone doesn't always tell the whole story. What kind of crack is it? How severe? What caused it?
That's where RoadBench comes in. It's a brand new dataset, like a giant scrapbook, filled with high-quality photos of road damage. But here's the kicker: each photo is paired with a detailed description, written in plain language. Imagine someone describing the damage to you over the phone, that's the kind of detail we're talking about. This is where the "multimodal" thing comes in, merging images (visual mode) with text (language mode).
Now, with this richer dataset, the researchers created RoadCLIP. Think of RoadCLIP like a super-smart AI that can "see" the road damage and "read" about it at the same time. It's like teaching a computer to not just see a crack, but to understand it.
How does RoadCLIP work its magic?
Disease-Aware Positional Encoding: Imagine RoadCLIP putting on special glasses that highlight specific areas of damage. It's not just seeing a crack, but understanding where that crack starts, stops, and how it spreads. Like a doctor understanding the progression of a disease.
Road Condition Priors: This is like feeding RoadCLIP extra information about roads. What are roads made of? What are the common causes of damage? This helps it make more informed decisions.
But here's where it gets even more interesting. Creating a massive dataset like RoadBench can be time-consuming and expensive. So, the researchers used a clever trick: they used another AI, powered by GPT (the same technology behind some popular chatbots), to automatically generate more image-text pairs. This boosted the size and diversity of the dataset without needing tons of manual labor. This is like asking an expert to write variations of descriptions for the same problem, enriching the learning materials.
So, why does this matter? Well, the results are impressive. RoadCLIP, using both images and text, outperformed existing systems that only use images by a whopping 19.2%! That's a huge leap forward.
Think about the implications:
For city planners and transportation departments: This could lead to more efficient and accurate road maintenance, saving time and money. Imagine autonomous vehicles automatically reporting damage in real-time.
For drivers: Safer roads mean fewer accidents and less wear and tear on our vehicles.
For AI researchers: RoadBench provides a valuable resource for developing more sophisticated multimodal AI systems.
"These results highlight the advantages of integrating visual and textual information for enhanced road condition analysis, setting new benchmarks for the field and paving the way for more effective infrastructure monitoring through multimodal learning."
This research opens up some fascinating questions:
Could this technology be adapted to detect other types of infrastructure damage, like cracks in bridges or corrosion on pipelines?
How can we ensure that the AI-generated text is accurate and unbiased, avoiding potential misinterpretations or skewed data?
RoadCLIP and RoadBench are exciting steps towards smarter, safer roads. It's a testament to the power of combining different types of information to solve real-world problems. What do you think, learning crew? Let's discuss!Credit to Paper authors: Xi Xiao, Yunbei Zhang, Janet Wang, Lin Zhao, Yuxiang Wei, Hengjia Li, Yanshu Li, Xiao Wang, Swalpa Kumar Roy, Hao Xu, Tianyang Wang
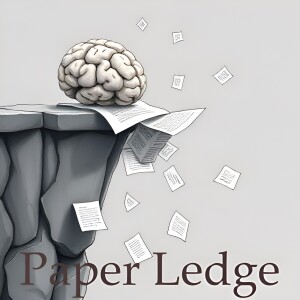
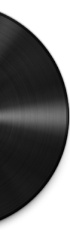
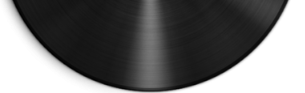
5 days ago
5 days ago
Hey PaperLedge learning crew, Ernis here, ready to dive into something really cool in the world of healthcare! Today, we're looking at a paper about using AI to help doctors diagnose eye diseases, specifically by looking at images of the back of your eye – what they call the fundus.
Now, imagine you're trying to teach a computer to be an eye doctor. It's not as simple as showing it a bunch of pictures. See, existing AI models, even the really big ones, struggle because the information they get is often fragmented. It's like giving a student only pieces of the puzzle without showing them the big picture. And sometimes, the computer's reasoning can be… well, a bit illogical from a doctor's point of view.
That's where this paper comes in. These researchers built something called FundusExpert – think of it as a specialized AI doctor for eyes! But it's not just the AI itself; they also created a new way to teach it, using something called FundusGen. FundusGen is like a super-detailed textbook with tons of eye images, but with a special twist.
FundusGen uses something called Fundus-Engine. Imagine a smart system that automatically points out potential problem spots in the eye image. It then uses AI to add detailed descriptions and connect everything – the overall picture, the specific spots, and even the tiniest details – to the potential diagnoses. It’s like drawing lines between all the clues to solve a mystery!
And here’s the kicker: FundusGen doesn't just show the AI what the problem is, it also shows why. It creates what they call a "clinically aligned cognitive chain." This is like showing the AI the doctor's thought process, the steps they take to reach a diagnosis. This helps the AI understand the reasoning behind the diagnosis, not just memorize a bunch of images.
The results? Incredible! FundusExpert, trained with FundusGen, was way better at answering questions about eye diseases than other AI models, even ones that are much, much bigger. In fact, it beat one model, the 40B MedRegA, by a whopping 26.6%!
"FundusExpert achieves the best performance in ophthalmic question-answering tasks, surpassing the average accuracy of the 40B MedRegA by 26.6%."
It also did a fantastic job at writing reports about the eye images, sounding much more like a real doctor than other AI tools like GPT-4o. The AI was able to maintain a 77% clinical consistency compared to GPT-4o at only 47.6%!
"It also excels in zero-shot report generation tasks, achieving a clinical consistency of 77.0%, significantly outperforming GPT-4o's 47.6%."
The researchers even discovered something interesting about how well the AI learns. They found that the better the quality of the training data (thanks to FundusGen's detailed explanations), the more efficiently the AI could learn. It’s like saying a student learns faster and better with a great teacher and a well-organized textbook!
So, why does this matter?
For patients: This could lead to faster and more accurate diagnoses of eye diseases, potentially saving your vision!
For doctors: This could be a powerful tool to assist in diagnosis, especially in areas where specialists are scarce. It could also help doctors stay up-to-date on the latest research.
For AI researchers: This shows a promising new approach to training AI in specialized fields, focusing on quality data and logical reasoning.
Now, a couple of things that popped into my head while reading this paper:
How do we ensure that these AI systems are used ethically and responsibly? What safeguards need to be in place to prevent misuse or bias?
Could this approach be applied to other areas of medicine, like diagnosing skin conditions or analyzing X-rays? What are the limitations of this method?
This is a really fascinating piece of research, and I'm excited to see where it goes. You can find a link to the paper and the project on GitHub (https://github.com/MeteorElf/FundusExpert) in the show notes. Let me know what you think, learning crew! What other questions does this raise for you?Credit to Paper authors: Xinyao Liu, Diping Song
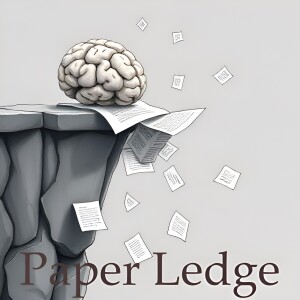
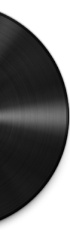
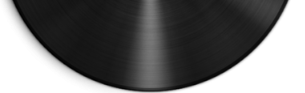
5 days ago
5 days ago
Hey PaperLedge learning crew, Ernis here, ready to dive into some seriously cool tech! Today, we're unpacking a paper about making data visualization, you know, those charts and graphs that help us understand information, way easier for everyone.
Now, let's be honest, creating a beautiful and informative chart can feel like trying to bake a soufflé without a recipe. It's tricky! You need design skills, you need to understand the software, and it can be a real headache. This paper tackles that problem head-on.
The researchers developed something called DataWink. Think of it as having a super-smart art director and data analyst combined into one handy tool. The core idea? Learning from existing, gorgeous visualizations.
Imagine you see a stunning infographic online. DataWink uses fancy AI, specifically what they call large multimodal models (LMMs) – basically a super-powered AI that can "see" and "understand" images and text – to figure out how that infographic was made. It breaks down the data, the colors, the shapes, everything!
"DataWink enables users to create custom visualizations by adapting high-quality examples."
It's like reverse-engineering a masterpiece, but instead of taking it apart, DataWink learns the secrets to its beauty.
Here’s the cool part: it creates a sort of "blueprint" of the visualization, a middle ground between the raw computer code that draws the shapes (called SVG) and the actual software that created it. This blueprint allows you to then take that blueprint and adapt it to your own data, your own story.
So, how do you actually use DataWink? Well, it’s all about conversation. You can tell the system what you want to change – maybe you want to highlight a specific trend, or use different colors to match your company's branding. You can even use simple widgets, like sliders and color pickers, to tweak the visual appearance.
It’s like having a conversation with a designer, but instead of endless email chains, you get instant visual feedback. You can adjust the data mapping – how the data is represented visually – and the design elements, all while keeping that original aesthetic quality that caught your eye in the first place.
Think of it like this: You find a beautiful dress online, but it's the wrong color and size. DataWink helps you "remake" the dress to fit you perfectly, using the original dress's design as a guide.
Now, does it actually work? The researchers put DataWink to the test with a user study. They had 12 people try it out, giving them tasks like recreating existing visualizations and exploring the system's capabilities. The results were pretty impressive.
People found DataWink easy to learn and effective for creating personalized visualizations. It seems like this example-driven approach really does help democratize visualization creation, making it accessible to more people.
Why does this matter?
For researchers: It opens up new avenues for exploring how AI can assist in creative tasks.
For businesses: It empowers employees to create compelling data visualizations without needing to hire expensive designers.
For educators: It provides a user-friendly tool for teaching data literacy and visual communication.
This paper really highlights the potential of AI to bridge the gap between complex tools and everyday users. It's about making technology more accessible and empowering people to tell their stories with data.
So, what do you think, learning crew? Does this approach truly "democratize" data visualization, or are there still limitations? And if everyone has access to tools like DataWink, will we see an explosion of beautiful (but maybe misleading) charts and graphs? Let's discuss!Credit to Paper authors: Liwenhan Xie, Yanna Lin, Can Liu, Huamin Qu, Xinhuan Shu
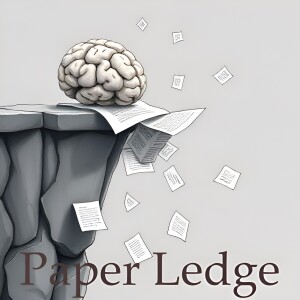
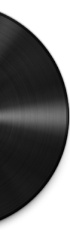
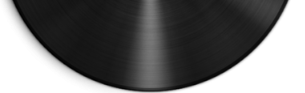
5 days ago
5 days ago
Alright learning crew, Ernis here, ready to dive into another fascinating paper! Today, we're talking about something super relevant in our AI-driven world: making AI characters, like the ones you might interact with in a game or even a customer service chatbot, really believable.
Think about it: you're playing a game, and you meet a character who's supposed to be, say, Sherlock Holmes. But they just...don't sound like him. They're missing that sharp wit, that keen observation, that distinctive way of speaking. It breaks the immersion, right?
That's the problem this paper tackles. Current AI models, even the really big and powerful ones called Large Language Models (LLMs), often struggle to truly embody a specific character. Just telling them "be Sherlock Holmes" isn't enough. It's like asking someone to impersonate Elvis just by hearing his name – you might get a vague impression, but not the King himself!
Now, one way to make AI better at this is to train it specifically on tons of Sherlock Holmes dialogue. But that's a huge undertaking! It requires a mountain of data and a lot of computer power. It's like teaching someone to cook by making them prepare hundreds of different dishes – effective, but time-consuming and expensive.
This is where the cool new technique, called Test-Time-Matching (TTM), comes in. It's a "training-free" approach, meaning it skips the massive training phase. Instead, it focuses on being clever in the moment, when the AI is actually interacting with you. Think of it like improv comedy: instead of memorizing a script, the AI learns to use its existing knowledge in a smart, character-specific way.
So, how does TTM work? Well, the researchers essentially figured out how to break down a character into three key ingredients:
Personality: What are their core traits? Are they grumpy, optimistic, logical, emotional?
Memory: What's their backstory? What important events have shaped them? This is the character's "history."
Linguistic Style: How do they speak? Do they use formal language, slang, metaphors, sarcasm? This is the character's "voice."
TTM then uses the LLM to automatically extract these features. It's like having an AI analyze Sherlock Holmes and figure out, "Okay, this guy is highly logical, remembers every tiny detail, and speaks in a very precise and analytical manner."
Once these ingredients are separated, TTM uses them in a three-step process to generate dialogue. It's like a recipe: first, add the personality; then, stir in the relevant memories; and finally, season with the perfect linguistic style. The result? An AI character that feels much more authentic and consistent.
The really impressive thing is that TTM allows you to mix and match these features. Want Sherlock Holmes with a slightly different personality, or speaking in a more modern way? TTM can do that! It's like being able to tweak the recipe to create your own unique version of the character.
The researchers tested TTM by having people interact with the AI characters and rate how well they captured the essence of the role. The results were fantastic! TTM consistently outperformed other methods in generating expressive and believable character dialogues.
Why does this matter? Well, for gamers, it means more immersive and engaging experiences. For educators, it could lead to more realistic and effective learning simulations. For anyone interacting with AI, it means more natural and human-like conversations. And for the creative crew out there, it could give you a great method for making characters for your stories.
"...our method achieves the outstanding performance in generating expressive and stylistically consistent character dialogues."
So, some questions that popped into my head: Could this technology be used to create convincing historical figures for interactive documentaries? And what are the ethical considerations of creating AI characters that are too realistic – could they be used to deceive or manipulate people?
This paper really opens up some exciting possibilities, and I'm eager to see where this research leads us. Let me know what you think learning crew!Credit to Paper authors: Xiaoyu Zhan, Xinyu Fu, Hao Sun, Yuanqi Li, Jie Guo, Yanwen Guo