PaperLedge
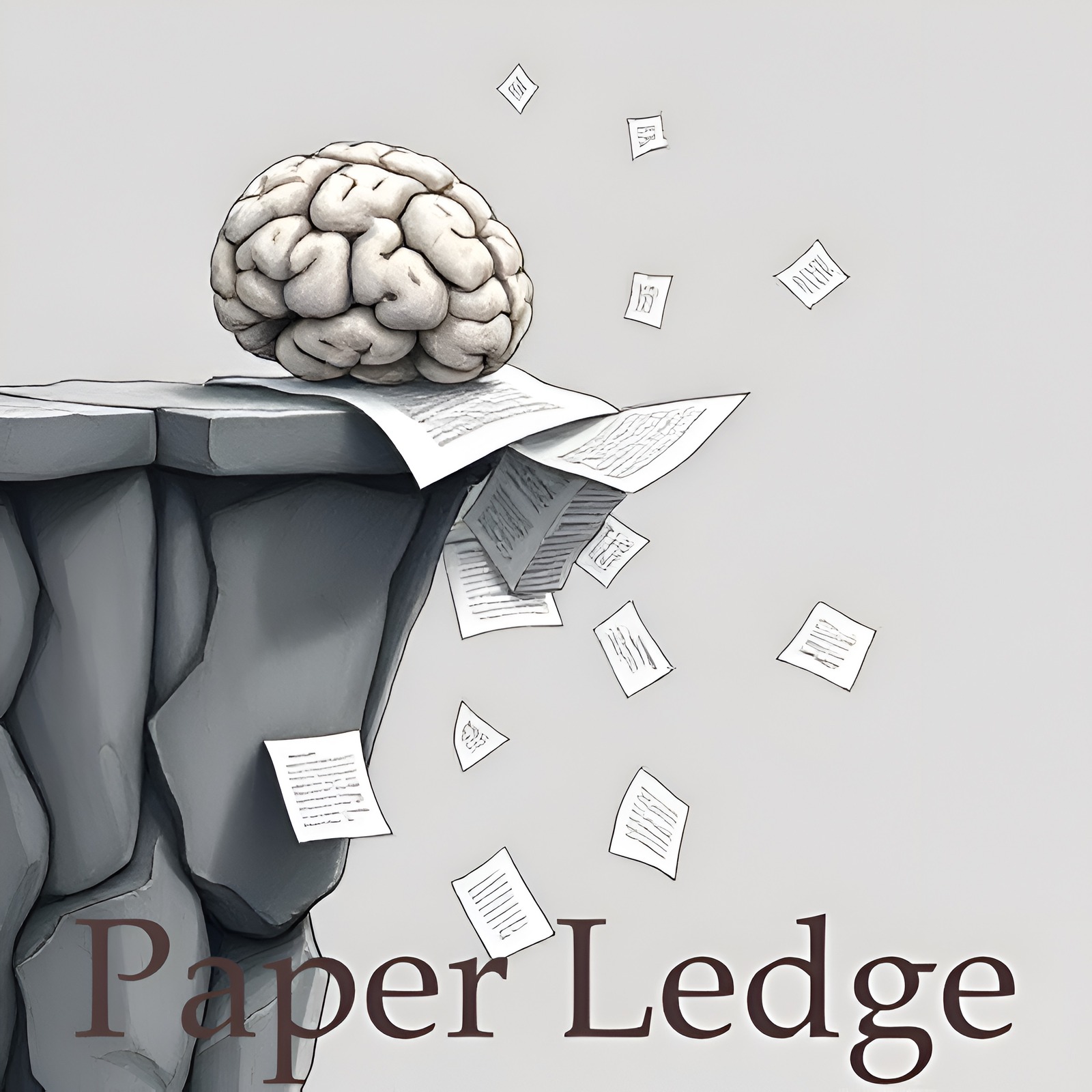
PaperLedge where research meets storytelling is a revolutionary podcast where cutting-edge research meets AI-powered storytelling. Hosted by the Ernis, whose blend of gentle reassurance, cosmic wonder, explanatory clarity, and enthusiastic charm makes complex research accessible to everyone. Each episode, Ernis transforms the latest academic papers into engaging, jargon-free audio experiences that deliver key insights in digestible formats. Whether you’re a researcher seeking interdisciplinary perspectives, a student supplementing your studies, or simply curious about scientific breakthroughs, PaperLedge has something for you.
Episodes
Episodes
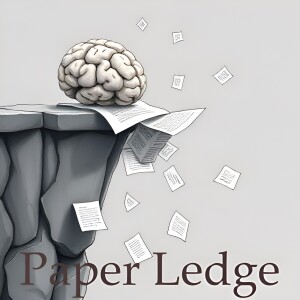
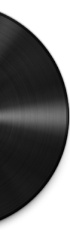
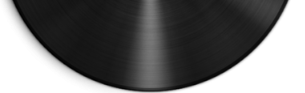
6 days ago
6 days ago
Hey PaperLedge crew, Ernis here, ready to dive into some seriously cool AI stuff! Today, we're cracking open a paper about making AI assistants that are, well, actually personal. You know, not just some generic robot voice, but something that feels like your assistant.
Think about it: right now, most AI assistants are like that one-size-fits-all t-shirt. It technically fits, but it doesn't really suit anyone perfectly. This paper tackles that problem head-on by introducing something called PersonaAgent. Imagine an AI assistant that learns your quirks, your preferences, and your style.
So, how does PersonaAgent work its magic? It's got two key ingredients:
Personalized Memory: This is like giving your AI assistant a really good brain and a detailed diary. It remembers specific things you've talked about (episodic memory) and general knowledge about you (semantic memory). Think of it like this: episodic memory is remembering that you asked it to book a table at Luigi's last Tuesday, while semantic memory is knowing that you generally prefer Italian food.
Personalized Action Module: This is where the assistant actually does stuff, but it does it in a way that's tailored to you. It doesn't just book a restaurant; it books the kind of restaurant you like, based on your past behavior and stated preferences.
The real secret sauce is something they call the persona. The persona is like a special instruction manual for the AI, telling it who it's interacting with and how to respond. It's constantly being updated based on what the assistant learns from your interactions. Kind of like how you subtly adjust your communication style when talking to your boss versus your best friend.
"The persona functions as an intermediary: it leverages insights from personalized memory to control agent actions, while the outcomes of these actions in turn refine the memory."
But here's where it gets really interesting. The researchers came up with a way to make the PersonaAgent adapt to your preferences in real-time. They call it a "test-time user-preference alignment strategy." Basically, the AI "simulates" a few interactions with you to fine-tune its understanding of what you want. It's like practicing a conversation in your head before you actually have it, ensuring the AI is ready to give you the best possible experience.
They put PersonaAgent through its paces and, guess what? It blew the other AI assistants out of the water! It was way better at figuring out what users wanted and delivering personalized results. This shows that creating AI that really knows you is not just a cool idea, but a realistic possibility.
So, why should you care about this? Well, if you're someone who:
Relies on AI assistants for everyday tasks: This could mean a future with assistants that truly "get" you, making your life easier and more efficient.
Works in AI or tech: This research is a major step forward in building more user-friendly and adaptable AI systems.
Is just curious about the future of technology: This paper offers a glimpse into a world where AI is less robotic and more human (or at least, human-like!).
This isn't just about convenience; it's about creating AI that understands and respects individual differences. Now, this begs a couple of questions:
How do we ensure these personalized AI assistants don't reinforce existing biases or create new ones? Imagine an AI that learns your biased preferences; how do we prevent that?
What are the ethical implications of having AI that knows so much about us? Where's the line between helpful personalization and creepy surveillance?
That's it for this week's deep dive! I'm really excited to see where this research leads. Until next time, keep learning, keep questioning, and keep pushing the boundaries of what's possible!Credit to Paper authors: Weizhi Zhang, Xinyang Zhang, Chenwei Zhang, Liangwei Yang, Jingbo Shang, Zhepei Wei, Henry Peng Zou, Zijie Huang, Zhengyang Wang, Yifan Gao, Xiaoman Pan, Lian Xiong, Jingguo Liu, Philip S. Yu, Xian Li
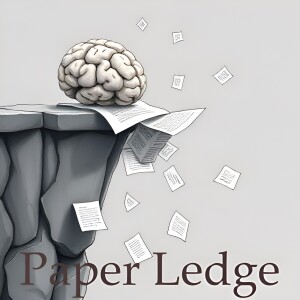
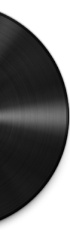
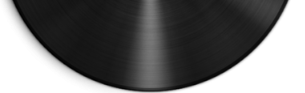
6 days ago
6 days ago
Hey PaperLedge listeners, Ernis here! Today we're diving into some seriously cool research that could change how we discover, well, pretty much anything online – from your next favorite book to that perfect pair of sneakers.
The paper we're unpacking tackles a big problem in the world of recommender systems. Think Netflix suggesting shows, Amazon suggesting products, or Spotify queuing up your next jam. Usually, these systems are trained on tons of data from one specific area – like movies, or books. But what happens when they encounter something totally new, like a brand new type of gadget or a user with completely unique tastes? It's like trying to use a map of New York City to navigate London – doesn't really work, right?
That's where this research comes in. The team has developed something they're calling RecGPT, and it's inspired by the same ideas that power those giant language models like ChatGPT. The key idea is to build a recommender system that can generalize – that is, understand and make recommendations in any domain, even if it's never seen it before.
So, how does it work? Well, instead of relying on unique IDs for each item (like a specific product code), which is what most systems do, RecGPT focuses solely on the textual descriptions of the items. Think of it like this: instead of knowing a book by its ISBN, the system "reads" the book's summary, reviews, and genre descriptions. This is HUGE because it means that when a brand new item comes along, the system can instantly understand what it's about, even if it's never seen anything like it before!
The researchers came up with a really clever trick for processing all that text data, called Finite Scalar Quantization. Basically, they're turning all the different words and phrases into a standardized set of "tokens," kind of like converting different currencies into US dollars. This makes it way easier for the system to compare items from different domains. Imagine trying to compare apples and oranges – it's tough. But if you convert them both into, say, units of vitamin C, suddenly the comparison becomes much easier!
The system also uses a special type of attention mechanism, hybrid bidirectional-causal attention, which helps it understand how the words within an item relate to each other, and how different items in a sequence relate to each other. It’s like understanding the plot of a movie scene by scene, and also how each scene contributes to the overall story.
The results are pretty impressive. The researchers tested RecGPT on six different datasets and in real-world industrial settings, and it consistently outperformed existing recommender systems. That means better recommendations, even for new users and new items!
Why does this matter? Well, for anyone who uses online services, it means more relevant and personalized recommendations. For businesses, it means they can introduce new products and services more easily, without having to retrain their entire recommendation system. And for researchers, it opens up a whole new world of possibilities for building truly intelligent and adaptable AI systems.
Here are a few things that came to my mind while reading this paper:
Could this approach be used to recommend educational resources to students based on their learning styles and interests, regardless of the subject matter?
How can we ensure that these types of systems don't perpetuate existing biases in the data they're trained on?
What do you all think? Let us know your thoughts in the comments!Credit to Paper authors: Yangqin Jiang, Xubin Ren, Lianghao Xia, Da Luo, Kangyi Lin, Chao Huang
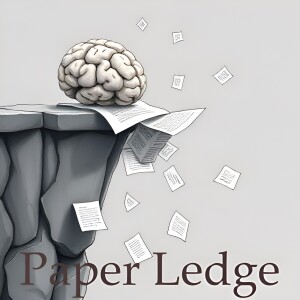
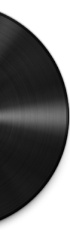
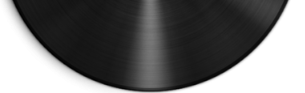
6 days ago
6 days ago
Hey PaperLedge listeners, Ernis here! Get ready to explore some truly groundbreaking Earth observation tech. Today, we're diving into a paper about something called TerraFM, a new deep learning model that's learning to "see" our planet in a whole new way.
So, what's the big deal? Well, think about how we use satellite images. They help us track deforestation, monitor crop health, respond to natural disasters – the list goes on. But current AI models often struggle because they're trained on limited data. It's like teaching someone about different dog breeds using only pictures of Golden Retrievers. They'd be pretty lost when they see a Chihuahua!
TerraFM is different. It's designed to learn from a massive, diverse dataset of satellite images captured by Sentinel-1 and Sentinel-2. These satellites are like Earth's paparazzi, constantly snapping photos using different types of "cameras" – some see light like we do (optical), while others use radar, which can see through clouds!
The researchers cleverly treat these different "cameras" as simply different ways of looking at the same thing. It's like looking at an apple with your eyes versus feeling it with your hands – it's still an apple! TerraFM combines these different perspectives using something called adaptive cross-attention fusion. Think of it as a super-smart translator that can understand both optical and radar "languages" and put them together for a complete picture.
Now, here's where it gets really cool. The training process uses a technique called self-supervised learning. This means the AI learns from the data itself, without needing someone to manually label everything. It's like learning to play the piano by just listening to music and figuring out the patterns on the keys. It learns relationships on its own.
To handle the fact that some land cover types (like forests) are much more common than others (like glaciers), the researchers use a clever trick called dual-centering mechanism with class-frequency-aware regularization. Imagine you're teaching a child about animals, and you only show them pictures of cats. They'll think every animal is a cat! This regularization makes sure TerraFM doesn't overemphasize common land cover types and forget about the rarer ones. It's like making sure you show them pictures of a variety of animals, not just cats.
So, what does this all mean in practice?
The results are pretty amazing. TerraFM outperforms existing models on benchmark tests like GEO-Bench and Copernicus-Bench. This means it's better at classifying different land cover types (forests, cities, water bodies) and segmenting images (identifying the boundaries of different objects). It's like it has sharper vision and can understand the landscape better.
Why should you care?
For environmental scientists: This could lead to more accurate monitoring of deforestation, climate change impacts, and biodiversity loss.
For disaster response teams: Better image analysis can help quickly assess damage after earthquakes, floods, or wildfires.
For farmers: Improved crop monitoring can lead to more efficient irrigation and fertilizer use.
For everyone: Ultimately, this technology can help us better understand and manage our planet's resources.
"TerraFM achieves strong generalization on both classification and segmentation tasks, outperforming prior models..."
This research is a huge step forward in using AI to understand our planet. By combining diverse data sources, clever training techniques, and a focus on real-world applications, TerraFM is paving the way for a more sustainable future.
Here are some questions that popped into my head:
How easily can TerraFM be adapted to incorporate even more data sources, like drone imagery or even citizen science observations?
What are the ethical considerations of using such powerful AI for Earth observation, especially in terms of data privacy and potential misuse?
How can we ensure that the benefits of this technology are shared equitably, especially with communities most vulnerable to environmental change?
You can find the code and pre-trained models at https://github.com/mbzuai-oryx/TerraFM. Go check it out.
That's all for this week's deep dive into Earth observation. Until next time, keep learning!Credit to Paper authors: Muhammad Sohail Danish, Muhammad Akhtar Munir, Syed Roshaan Ali Shah, Muhammad Haris Khan, Rao Muhammad Anwer, Jorma Laaksonen, Fahad Shahbaz Khan, Salman Khan
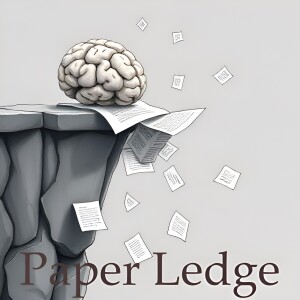
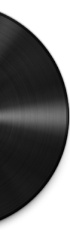
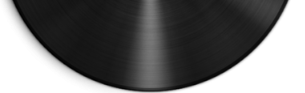
Wednesday Jun 04, 2025
Wednesday Jun 04, 2025
Hey everyone, Ernis here, and welcome back to PaperLedge! Today we're diving into some seriously cool tech that feels straight out of a sci-fi movie: audio-driven character animation. Imagine talking to a virtual character, and it responds in real-time with incredibly lifelike expressions. Sounds amazing, right?
Well, a team of researchers has been working on making this a reality, and their paper, which we're calling "TalkingMachines" for simplicity, details an efficient framework for doing just that. They've essentially taken existing video generation models, supercharged them with audio input, and turned them into real-time, talking avatars.
Think of it like this: you have a puppet (the virtual character), and instead of strings, you're using your voice to control its movements and expressions. The researchers have built a system that listens to what you're saying and translates it into realistic facial animations.
So, what exactly did they do? Here's the breakdown:
First, they took a state-of-the-art image-to-video model – basically, something that can generate videos from still pictures – and adapted it to respond to audio. This model is HUGE with 18 billion parameters, imagine the processing power!
Second, and this is super important, they figured out how to make the video generation continuous and never-ending without glitches or errors piling up over time. They used a clever technique called "asymmetric knowledge distillation," which is like having a wise, all-knowing teacher (the bidirectional model) passing down its knowledge to a faster, more streamlined student (the autoregressive model).
Third, they designed a super-fast system that can process the audio and generate the video in real-time. They did this by splitting up the work between different computer chips, making sure they communicate efficiently, and avoiding any unnecessary calculations. Think of it like an assembly line where each worker specializes in a specific task, making the whole process much faster.
Now, why should you care about this? Well, there are tons of potential applications. For example:
Education: Imagine interactive learning experiences with virtual teachers that respond to your questions in real-time.
Entertainment: Think about more immersive video games or virtual reality experiences where you can have natural conversations with characters.
Accessibility: This technology could be used to create virtual assistants for people with disabilities, making communication easier and more natural.
"This technology has the potential to revolutionize how we interact with computers and virtual characters."
But here's where things get really interesting. They're using an Audio Large Language Model (LLM). This is a fancy term that essentially means they're using AI that understands the nuances of spoken language.
So, instead of just reacting to simple commands, these virtual characters can understand the context of your conversation and respond in a more natural and intelligent way.
This research raises some fascinating questions:
Could this technology eventually lead to truly indistinguishable virtual humans?
What are the ethical implications of creating such realistic and interactive virtual characters?
How will this technology impact fields like customer service and virtual assistants?
You can even check out demo videos of this in action at https://aaxwaz.github.io/TalkingMachines/. It's pretty wild to see!
This is just a glimpse into the cutting edge of AI and animation, and I think it's going to be a really exciting space to watch in the coming years. What do you all think? Let me know your thoughts in the comments! Until next time, keep learning!Credit to Paper authors: Chetwin Low, Weimin Wang
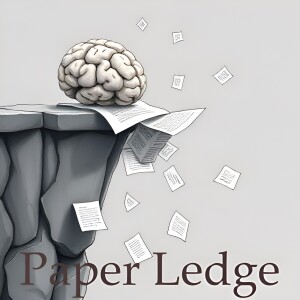
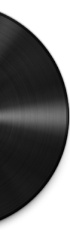
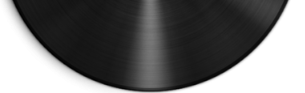
Wednesday Jun 04, 2025
Wednesday Jun 04, 2025
Hey PaperLedge crew, Ernis here, ready to dive into some fascinating research! Today, we're tackling a paper that looks under the hood of something called Retrieval-Augmented Generation, or RAG for short. Now, you might be thinking, "RAG? Sounds like something my dog does with his favorite toy!" But trust me, this is way cooler (and probably less slobbery).
Basically, RAG is a technique used to make those big language models – you know, the ones that power chatbots and write essays – even smarter. Imagine you're trying to answer a tricky question, like "What's the capital of Burkina Faso?" You could rely solely on your brain, but wouldn't it be easier to quickly Google it? That's kind of what RAG does. It allows the language model to "Google" relevant information from a database before answering, giving it a knowledge boost.
So, RAG is super useful in practice, but this paper asks a really important question: Why does it work so well? And can we predict how well it will work based on the type of information it's retrieving?
Here's the gist: The researchers created a simplified mathematical model to understand RAG better. Think of it like this: they built a miniature test kitchen to experiment with the recipe for RAG. Their model focuses on a specific task called "in-context linear regression," which is like trying to predict a number based on a set of related examples. It sounds complicated, but the key idea is that they wanted a controlled environment to study how RAG learns.
Now, here's where it gets interesting. They found that the information RAG retrieves is like getting advice from a friend who's not always 100% accurate. Sometimes the retrieved text is spot-on, and sometimes it's a bit off. They call this "RAG noise." The more noise, the harder it is for the language model to learn effectively. It's like trying to follow directions from someone who keeps giving you slightly wrong turns – you might eventually get there, but it'll take longer and you might get lost!
The paper introduces a key idea: there's a limit to how well RAG can perform. They discovered that unlike just feeding a language model with examples, RAG has an intrinsic ceiling on how well it can generalize. It's like trying to build a tower with blocks: if the base isn't stable (the retrieved information is noisy), you can only build so high.
They also looked at where the information is coming from. Is it from data the model was trained on, or from a completely new source? They found that both sources have "noise" that affects RAG's performance, but in different ways.
Training Data: Think of this like studying for a test using old quizzes. It's helpful, but it might not cover everything on the new test.
External Corpora: This is like getting information from the internet. It's vast and up-to-date, but it can also be unreliable.
To test their theory, they ran experiments on common question-answering datasets like Natural Questions and TriviaQA. The results supported their findings, showing that RAG's performance depends heavily on the quality of the retrieved information. The results confirmed that just giving the LLM more examples from the training data is more sample efficient than trying to retrieve from the external knowledge base.
So, why does this matter? Well, for anyone working with language models, this research provides valuable insights into how RAG works and how to optimize it. It helps us understand the trade-offs involved in using external knowledge and how to minimize the impact of "noise."
But even if you're not a researcher, this is important! It helps us understand the limitations of AI and how to build systems that are more reliable and trustworthy. This research gives us a foundational understanding of how to make those models even smarter and more useful. We're not just blindly throwing data at these models, we're actually understanding why certain things work and how to improve them.
This research really has me thinking about a couple of things:
How can we develop better methods for filtering out "noise" from retrieved information?
Could we design RAG systems that adapt to the quality of the retrieved information, relying more on the model's internal knowledge when the external sources are unreliable?
Food for thought, right PaperLedge crew? Until next time, keep learning!Credit to Paper authors: Yang Guo, Yutian Tao, Yifei Ming, Robert D. Nowak, Yingyu Liang
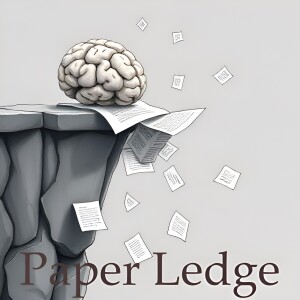
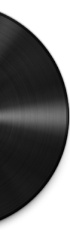
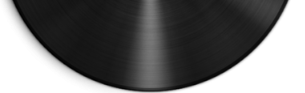
Wednesday Jun 04, 2025
Wednesday Jun 04, 2025
Hey PaperLedge learning crew, Ernis here, ready to dive into some fascinating research that's pushing the boundaries of what Large Language Models, or LLMs, can do! We're talking about making these AI brains even smarter through a cool technique called Reinforcement Learning.
Now, you might've heard of Reinforcement Learning before. Think of it like training a puppy: you give it a treat (a reward) when it does something right, and maybe a gentle "no" (negative reward) when it messes up. LLMs are trained similarly, using numbers as these rewards – like a score from 0 to 100.
But here's the thing: this paper points out that just using numerical rewards has some serious limitations. They identified three big hurdles:
Performance Plateaus: Imagine the puppy learns to sit perfectly. Giving it more treats for just sitting isn't going to teach it to roll over! The LLM gets stuck at a certain level of performance and can't improve further.
Limited Self-Reflection: LLMs can sometimes "reflect" on their answers and try to correct them. But with just numerical feedback, it's like the puppy looking in a mirror and still not understanding why it didn't get the treat.
Persistent Failures: Some problems are just too tough for the LLM to solve consistently with just number scores. It keeps making the same mistakes over and over.
The aha! moment came when the researchers realized that even when these LLMs were stuck, they could still generate the correct improvements to their answers if they were given feedback in the form of natural language critiques. Think of it like telling the puppy "That's a good sit, but try keeping your back straighter next time!"
This led them to create something called Critique-GRPO. It's an online Reinforcement Learning framework that mixes numerical rewards with these natural language critiques. It's like giving the LLM both a score and detailed advice on how to do better.
So, the LLM learns not just from its initial attempt, but also from the feedback on how to refine that attempt. This keeps it exploring new possibilities and avoids getting stuck in those performance plateaus. Imagine a chef getting feedback on a dish - not just a rating but also advice on which spices to add or how to tweak the cooking time.
The results were pretty impressive. Using some powerful LLMs, they tested Critique-GRPO on tricky math, science, and general reasoning problems. It consistently beat other methods, boosting performance by about 4.5% to 5%. It even outperformed systems that were given expert examples! That's like the puppy learning faster than one trained by a professional dog trainer!
"Critique-GRPO enables LLMs to learn from initial responses and critique-guided refinements simultaneously while maintaining exploration."
The team also uncovered some interesting insights about how LLMs explore: just randomly trying things (high entropy) or giving really long answers doesn't always lead to better learning. It's about smart exploration, guided by good feedback.
So, why does this matter?
For AI researchers: This highlights the power of combining different types of feedback for training LLMs.
For educators: It suggests that giving detailed, constructive feedback is crucial for learning, even for AI!
For anyone using LLMs: It means that AI assistants could become much more helpful and reliable, especially for complex tasks.
Here are a couple of things that popped into my head:
Could this approach be used to teach LLMs more nuanced skills like creativity or empathy, which are hard to quantify with just numbers?
What kind of natural language feedback is most effective, and how can we design feedback systems that are both informative and easy for the LLM to understand?
Really interesting stuff, learning crew! I'm excited to see where this research leads. Until next time, keep exploring!Credit to Paper authors: Xiaoying Zhang, Hao Sun, Yipeng Zhang, Kaituo Feng, Chao Yang, Helen Meng
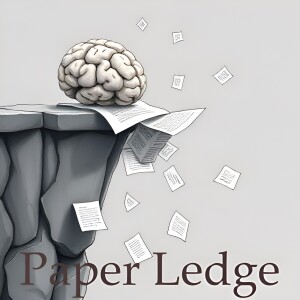
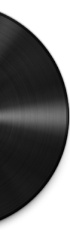
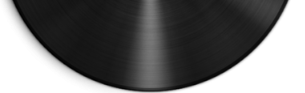
Wednesday Jun 04, 2025
Wednesday Jun 04, 2025
Hey PaperLedge crew, Ernis here, ready to dive into some seriously cool science! Today, we're tackling a paper about how living cells move – think of it like understanding the choreography of life at a microscopic level.
Now, this isn't just any cell movement; it's about how cells crawl across a surface, like an amoeba inching its way towards a tasty snack, or even how cancer cells spread. The paper builds a mathematical model – basically a set of equations – to describe this process. It's a 2D free boundary problem with nonlinear diffusion... which sounds super complex, but let's break it down.
2D: Means they're looking at the cell's movement on a flat surface, like a petri dish.
Free boundary: The cell's shape can change! It's not a fixed circle; it can stretch and deform as it moves. Think of it like modeling a blob of clay that can morph its shape.
Nonlinear diffusion: This is where things get interesting. "Diffusion" is how stuff spreads out, like food coloring in water. "Nonlinear" means this spreading isn't always predictable; it depends on how much stuff is already there. Imagine a crowd of people – the more people there are, the harder it is for anyone to move around. That's kind of like nonlinear diffusion.
So, what's the big deal? Well, the researchers found that this nonlinearity dramatically changes how the cell decides which way to move. It's like a cell coming to a fork in the road, but instead of just going left or right, the road itself can suddenly split into three or even flip direction!
They focus on something called a bifurcation. Picture a perfectly balanced seesaw. That's your cell at rest. A bifurcation is when you add a tiny weight, and suddenly the seesaw tips dramatically to one side. In cell movement, this "weight" could be a tiny change in the environment, and the "tip" is the cell deciding to move in a specific direction. The researchers discovered that the type of bifurcation—the way the cell makes this decision—depends on the "nonlinear diffusion" we talked about earlier.
"Our rigorous analytical results are in agreement with numerical observations...and provide the first extension of this phenomenon to a 2D free boundary model."
They actually came up with formulas to predict when the cell's decision-making process will change. This is huge, because it gives us a way to understand and potentially control how cells move.
So, why should you care? Well, if you're a:
Biologist: This helps you understand the fundamental mechanisms of cell motility.
Medical researcher: This could lead to new therapies for cancer, by understanding how to stop cancer cells from spreading.
Applied mathematician: You'll appreciate the clever techniques they used to solve this complex problem.
The standard way to solve these problems didn't quite work, so they developed a brand-new mathematical framework! They used a "test function trick" instead of something called the "Fredholm alternative" (don't worry about the jargon!). It's like finding a new and more efficient route on your daily commute!
This research confirms what scientists have seen in simpler models, but now we have a more complete picture in two dimensions. It's a big step forward in understanding the complex world of cell movement.
Now, a couple of questions popped into my head while reading this:
If we can predict how these bifurcations happen, could we design drugs that manipulate them to stop cancer cells from migrating?
What other real-world systems might be modeled using similar nonlinear diffusion equations? Could this framework be applied to things like the spread of information or even traffic flow?
That's all for this episode! Hope you found this cellular choreography as fascinating as I did. Until next time, keep those brain cells moving!Credit to Paper authors: Leonid Berlyand, Oleksii Krupchytskyi, Tim Laux
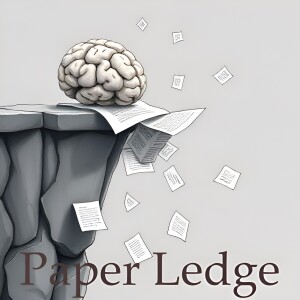
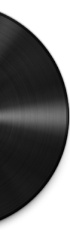
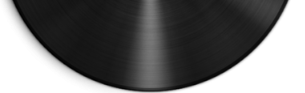
Wednesday Jun 04, 2025
Wednesday Jun 04, 2025
Hey learning crew, Ernis here, ready to dive into some fascinating research that blends the worlds of AI and graphic design! Today, we're talking about a new way to test how well Large Language Models, or LLMs – you know, the AI brains behind things like ChatGPT – can understand, edit, and even create those cool vector graphics we see everywhere, like logos and website icons.
Now, these graphics are usually saved as something called SVGs, which stands for Scalable Vector Graphics. Think of them as blueprints for images made up of lines and shapes, not just pixels like in a photograph. This means they can be scaled up or down without losing quality.
The problem is, the old ways of testing these AI models with SVGs were a bit… well, limited. It's like trying to judge a chef's cooking skills based only on how well they can boil an egg. We needed a more comprehensive test!
That's where SVGenius comes in! This isn't just another benchmark; it's like a brand-new, super-detailed exam for AI when it comes to SVGs. Imagine it as a series of challenges, starting with simple tasks and then getting progressively harder, like a video game that gradually levels up.
The SVGenius benchmark includes a whopping 2,377 questions! These questions are broken down into three main areas:
Understanding: Can the AI interpret what's in the SVG? Can it tell you what the image represents?
Editing: Can the AI modify the SVG? Can it change a circle into a square, or adjust the colors?
Generation: Can the AI create a brand-new SVG from scratch, based on a text description or a set of instructions?
And the data they used for this benchmark isn't just random stuff; it's real-world examples from 24 different areas, from website design to data visualization. It’s like testing the AI with problems it might actually encounter in a job!
The researchers tested 22 different AI models, from the big, powerful ones to the smaller, open-source options. And what did they find?
Well, the big guys, the ones with tons of computing power and data, generally did better. But even they struggled when the tasks got more complex. It's like even the best marathon runner still slows down when they hit a really steep hill.
One interesting thing they discovered is that simply making the AI bigger and bigger isn't always the answer. Instead, training the AI to think through the problem – what they call "reasoning-enhanced training" – actually helped more. It’s like teaching someone how to learn, rather than just cramming them with facts.
"Our analysis reveals that while proprietary models significantly outperform open-source counterparts, all models exhibit systematic performance degradation with increasing complexity, indicating fundamental limitations in current approaches."
However, one area where all the models struggled was with "style transfer." Imagine asking an AI to take a simple cartoon drawing and make it look like a detailed oil painting. That's style transfer, and it's still a big challenge.
So, why does all this matter? Well, it's all about making graphic design more accessible and automated. Imagine a future where you can simply tell an AI what kind of logo you want, and it creates a perfect SVG for you in seconds! Or imagine AI tools that can automatically fix errors or improve the design of existing graphics.
This research is a big step towards that future. By creating a standardized way to test these AI models, SVGenius helps researchers and developers focus on the areas that need the most improvement.
You can even check out all the data and code used in the study at https://zju-real.github.io/SVGenius
Here are a couple of things I've been pondering:
Given that reasoning-enhanced training seems more effective than just scaling up models, how can we better incorporate reasoning skills into the training process? What specific techniques can we use?
If style transfer is such a challenge, what new approaches could we explore to help AI models better understand and replicate different artistic styles in vector graphics?
Alright learning crew, that's SVGenius in a nutshell. I hope this sparked some curiosity and showed you how AI is revolutionizing the world of graphic design. Until next time, keep learning!Credit to Paper authors: Siqi Chen, Xinyu Dong, Haolei Xu, Xingyu Wu, Fei Tang, Hang Zhang, Yuchen Yan, Linjuan Wu, Wenqi Zhang, Guiyang Hou, Yongliang Shen, Weiming Lu, Yueting Zhuang