PaperLedge
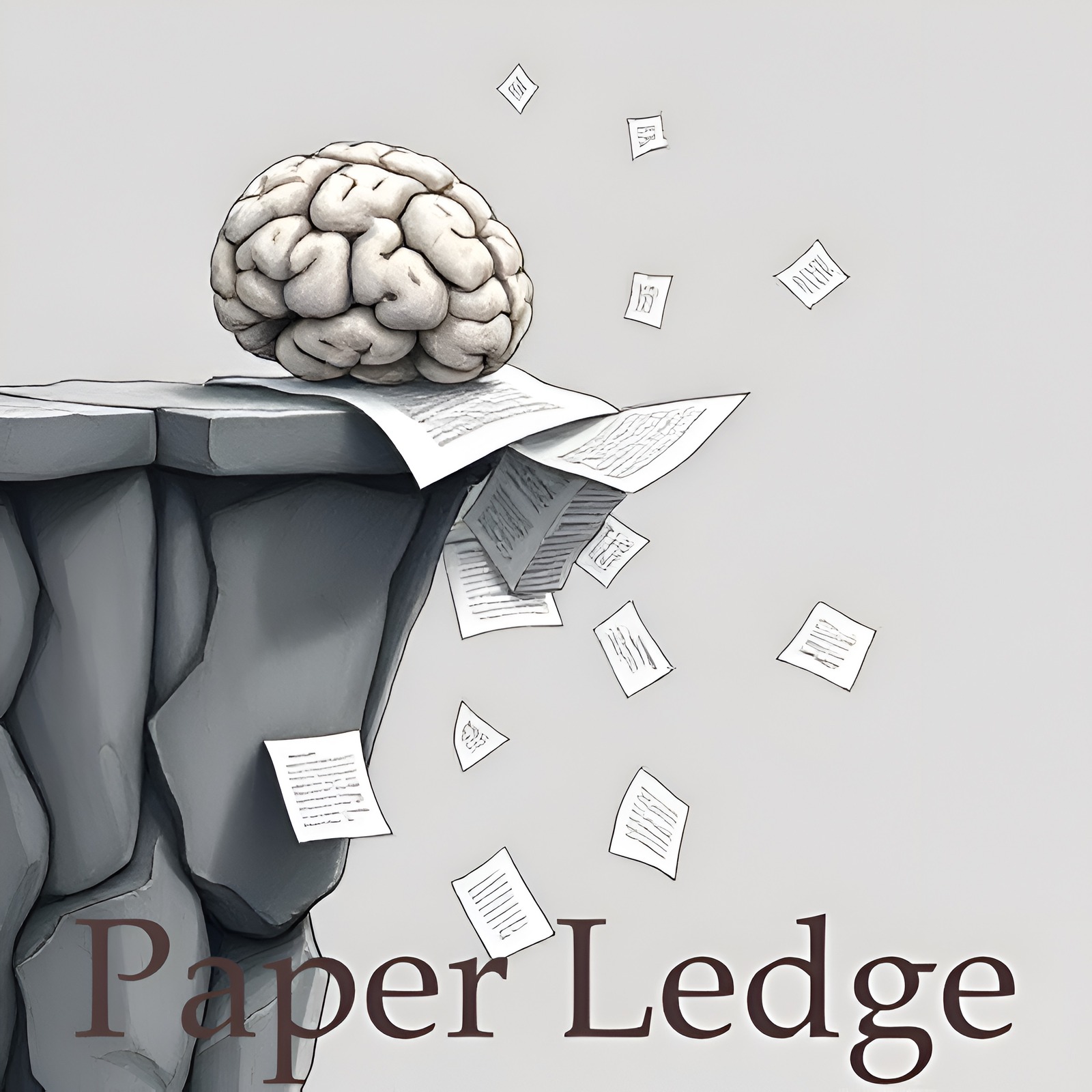
PaperLedge where research meets storytelling is a revolutionary podcast where cutting-edge research meets AI-powered storytelling. Hosted by the Ernis, whose blend of gentle reassurance, cosmic wonder, explanatory clarity, and enthusiastic charm makes complex research accessible to everyone. Each episode, Ernis transforms the latest academic papers into engaging, jargon-free audio experiences that deliver key insights in digestible formats. Whether you’re a researcher seeking interdisciplinary perspectives, a student supplementing your studies, or simply curious about scientific breakthroughs, PaperLedge has something for you.
Episodes
Episodes
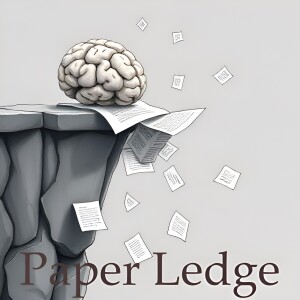
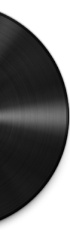
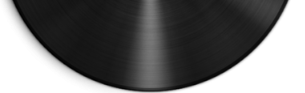
6 days ago
6 days ago
Hey PaperLedge crew, Ernis here, ready to dive into some cutting-edge AI research! Today, we're tackling a paper all about keeping AI agents safe and secure as they learn to work together. Think of it like this: imagine you have a team of super-smart robots, each with a special skill. You want them to collaborate on a project, right? But how do you make sure they don't accidentally mess things up, or worse, get hacked?
That's where protocols like Google's Agent2Agent, or A2A for short, come in. These protocols are like the rules of the road for AI agents, ensuring they can communicate and collaborate effectively. This paper takes a deep dive into the security aspects of A2A, and the core idea is that as AI agents become more complex and work together more often, it's absolutely vital that we understand how to keep those interactions secure.
The researchers started by breaking down A2A into its core components – like looking under the hood of a car to see how all the parts work. They then used a framework called MAESTRO, specifically designed for AI risks, to proactively find potential security holes. Think of MAESTRO as a security checklist for AI, helping us identify vulnerabilities before they become problems.
They focused on key areas like how agents identify each other (Agent Card management), how to make sure tasks are carried out correctly (task execution integrity), and how agents prove they are who they say they are (authentication methodologies). It's like making sure each robot has a valid ID badge, follows the instructions precisely, and can prove it's not an imposter.
"Understanding the secure implementation of A2A is essential."
Based on their analysis, the researchers offer practical advice for developers. They recommend secure development methods and architectural best practices to build strong and reliable A2A systems. They even explored how A2A can work with another protocol, the Model Context Protocol (MCP), to further enhance security. It's like adding extra layers of protection to a fortress!
So, why does this research matter?
For developers: This paper provides practical guidance on how to build secure AI systems that can collaborate effectively.
For businesses: Understanding A2A security can help ensure that AI-powered processes are reliable and trustworthy.
For everyone: As AI becomes more integrated into our lives, ensuring its security is crucial for maintaining trust and preventing potential misuse.
Ultimately, this paper equips developers and architects with the knowledge needed to use the A2A protocol confidently, building the next generation of secure AI applications.
This research really got me thinking about a few things:
How can we ensure that AI agents are not only secure but also ethical in their interactions?
As AI systems become more autonomous, how do we maintain human oversight and prevent unintended consequences?
What role will governments and regulatory bodies play in shaping the development and deployment of secure AI protocols?
These are just a few of the questions that come to mind when we start talking about the security of collaborative AI agents. What are your thoughts, PaperLedge crew? Let's keep the conversation going!Credit to Paper authors: Idan Habler, Ken Huang, Vineeth Sai Narajala, Prashant Kulkarni
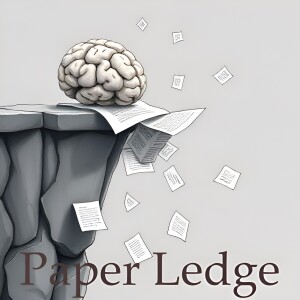
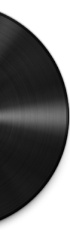
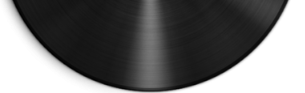
6 days ago
6 days ago
Hey Learning Crew, Ernis here, ready to dive into another fascinating paper! Today, we're tackling something super relevant to our increasingly digital world: spotting AI-generated text. Think of it like this: we're becoming detectives in the age of artificial intelligence!
So, why is this important? Well, imagine someone using AI to write essays for school, spreading fake news online, or even creating misleading marketing campaigns. It's a big deal! That's why researchers are working hard to develop tools that can tell the difference between text written by a human and text cranked out by a machine.
Now, this particular paper introduces a new framework called COT Fine-tuned. It's like a super-smart AI detective that not only figures out if a text was written by AI, but also tries to pinpoint which AI model was used! Think of it like identifying the brand of a car just by looking at the tire tracks.
The cool thing about COT Fine-tuned is that it uses something called Chain-of-Thought reasoning. Instead of just spitting out an answer, it actually explains its thinking process. It's like the detective showing you the clues they found and how they pieced them together. This makes the whole process more transparent and easier to understand. It's not just a black box; we get a peek inside!
To break it down, the system tackles two key tasks:
Task A: Is this text human-written or AI-generated? (The basic "is it AI?" question)
Task B: If it's AI-generated, which AI model wrote it? (The "which brand of AI?" question)
According to the paper, COT Fine-tuned is really good at both of these tasks. It's accurate in identifying AI-generated text and in figuring out which language model was behind it. Plus, the researchers showed that the Chain-of-Thought reasoning is actually a key part of what makes it so effective. It's not just about getting the right answer; it's about understanding why the answer is right.
"Our experiments demonstrate that COT Fine-tuned achieves high accuracy in both tasks, with strong performance in LLM identification and human-AI classification."
So, why should you care? Well, if you're a student, this kind of technology could help ensure academic integrity. If you're a journalist or someone who cares about accurate information, it could help you spot and debunk misinformation. And if you're working in the AI field, it can help you build more responsible and transparent AI systems.
This research is important because it's a step towards creating a world where we can trust the information we consume. It's about understanding the source and being able to verify the authenticity of content.
Here are a couple of things this paper made me wonder about:
How well does COT Fine-tuned work against new, previously unseen AI models? Is it constantly playing catch-up?
Could AI be used to intentionally create text that tricks these detectors? Are we in for an AI arms race?
What do you think, Learning Crew? Let me know your thoughts in the comments!Credit to Paper authors: Shifali Agrahari, Sanasam Ranbir Singh
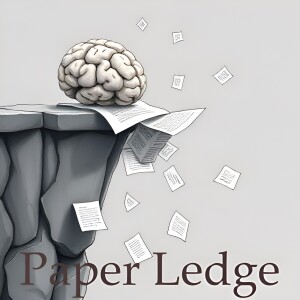
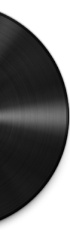
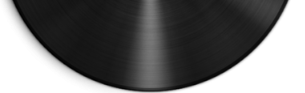
6 days ago
6 days ago
Hey PaperLedge listeners, Ernis here, ready to dive into some seriously cool research! Today, we're talking about a new AI system that's tackling a really tricky problem: optimization.
Now, optimization might sound super technical, but you're doing it all the time! Imagine you're planning a road trip. You want to find the best route, balancing things like distance, gas costs, and maybe even scenic views. That's optimization in action!
But for scientists and engineers, optimization problems can get incredibly complex. They might involve designing the most efficient airplane wing, or figuring out how to allocate resources in a supply chain. The hard part is turning a real-world problem into a precise mathematical equation that a computer can solve. That's where OptimAI comes in.
This research introduces OptimAI, a new system that uses the power of Large Language Models (LLMs) – think of them as super-smart AI text generators – to automatically solve optimization problems described in plain English. The researchers found that OptimAI beats existing methods by a significant margin.
Here's how it works, and it's pretty clever:
First, there's the Formulator. This AI agent takes the natural language description of the problem and translates it into a formal mathematical equation. Think of it as a translator between human language and math language.
Next, we have the Planner. This agent comes up with a high-level strategy for solving the problem before any calculations are done. It's like drawing up a blueprint before starting construction.
Then come the Coder and Code Critic. The Coder writes the actual computer code to solve the problem, while the Code Critic checks the code for errors and suggests improvements. They work together like a coding team, constantly refining the solution.
The researchers found that all four of these roles are crucial. If you take away the Planner or the Code Critic, the system's effectiveness drops dramatically. It's like trying to build a house without an architect or a quality inspector!
Here's a fun analogy: Imagine OptimAI is a team of chefs trying to create the perfect dish. The Formulator figures out what ingredients are available, the Planner decides on the overall recipe, the Coder actually cooks the dish, and the Code Critic tastes it and suggests improvements.
What's really interesting is that OptimAI uses something called UCB-based debug scheduling. This is a fancy way of saying that the system can dynamically switch between different solution plans if it detects a problem. It's like having a backup recipe in case the first one doesn't work out!
The research emphasizes the importance of teamwork. By combining different AI models within one system, OptimAI achieves impressive results. In fact, it achieved significantly higher accuracy on benchmark datasets compared to previous methods.
So, why does this research matter? Well, for scientists and engineers, OptimAI could save them a huge amount of time and effort in formulating and solving optimization problems. It could also lead to better solutions in areas like logistics, manufacturing, and even drug discovery.
But even if you're not a scientist, this research shows the potential of AI to tackle complex real-world problems. It highlights the power of collaboration, both between humans and machines, and between different AI agents.
Here are a few questions that popped into my head:
Could OptimAI eventually be used to solve everyday optimization problems, like planning the most efficient grocery shopping route or managing personal finances?
How can we ensure that AI systems like OptimAI are used ethically and responsibly, especially when they're applied to complex and sensitive problems?
What are the limits of this approach? Are there certain types of optimization problems that OptimAI just can't handle?
That's all for today's episode, folks! I hope you found this deep dive into OptimAI as fascinating as I did. Until next time, keep exploring!Credit to Paper authors: Raghav Thind, Youran Sun, Ling Liang, Haizhao Yang
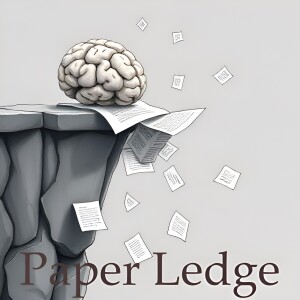
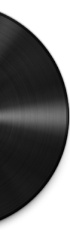
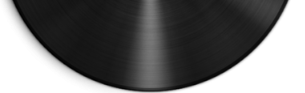
6 days ago
6 days ago
Hey everyone, Ernis here, and welcome back to PaperLedge! Today, we're diving into a fascinating paper that tackles a HUGE problem in the world of AI: how do we actually know if these fancy Large Language Models – LLMs for short – are any good, especially when it comes to languages other than English?
Think of it like this: Imagine you're teaching a parrot to speak. You can easily test its English vocabulary, right? But what if you wanted it to speak Spanish, Portuguese, or even Basque? Suddenly, finding reliable ways to test its understanding becomes a lot harder. That's the challenge these researchers are addressing.
Most of the tests and leaderboards we use to rank LLMs are heavily focused on English. It's like judging the parrot's overall intelligence solely on its English skills. These tests also tend to focus on basic language skills, rather than the kinds of tasks businesses and organizations actually need these models to perform. And, once a test is created, it usually stays the same for a long time.
That's where IberBench comes in. These researchers created a brand-new benchmark – essentially a comprehensive test – specifically designed to evaluate LLMs on languages spoken across the Iberian Peninsula (think Spain and Portugal) and Ibero-America.
Now, what makes IberBench so special? Well, a few things:
It's diverse: IberBench includes a whopping 101 datasets covering 22 different types of tasks. We're talking everything from figuring out the sentiment of a text (is it positive or negative?) to detecting toxic language online, to even summarizing long articles.
It's practical: It focuses on tasks that are actually useful in the real world, not just academic exercises.
It's dynamic: Unlike those static tests I mentioned earlier, IberBench is designed to be constantly updated and improved by the community. Think of it as a living, breathing evaluation system.
The researchers then put 23 different LLMs, ranging in size from tiny to pretty darn big, through the IberBench wringer. And the results? Some interesting insights:
"LLMs perform worse on industry-relevant tasks than in fundamental ones."
This means that even if an LLM is great at basic language skills, it might struggle with tasks that are actually useful for businesses. It also turns out that some languages, like Galician and Basque, consistently see lower performance. And, shockingly, some tasks were basically a coin flip for the LLMs – they performed no better than random chance! For other tasks, LLMs did okay, but they still weren't as good as systems specifically designed for those tasks.
So, why does this matter? Well, for a few reasons:
For developers: IberBench provides a valuable tool for building and improving LLMs for these languages. It highlights where models are strong and where they need work.
For businesses: If you're thinking about using LLMs to automate tasks in Spanish, Portuguese, or other Iberian/Ibero-American languages, IberBench can help you choose the right model for your needs.
For everyone: By creating a more diverse and representative benchmark, IberBench helps to ensure that AI benefits everyone, not just English speakers.
And the best part? The entire evaluation pipeline, from the datasets to the leaderboard, is open-source. That means anyone can use it, contribute to it, and help make it even better!
So, what do you think, learning crew? Here are a couple of questions that popped into my head:
Given these results, what are the ethical implications of deploying LLMs in languages where their performance is significantly lower?
How can we encourage more community involvement in developing and maintaining benchmarks like IberBench?
I'm excited to hear your thoughts on this one. Until next time, keep learning!Credit to Paper authors: José Ángel González, Ian Borrego Obrador, Álvaro Romo Herrero, Areg Mikael Sarvazyan, Mara Chinea-Ríos, Angelo Basile, Marc Franco-Salvador
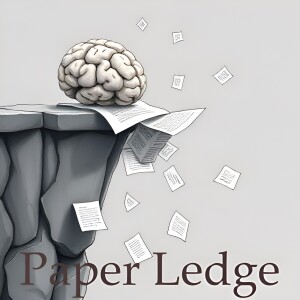
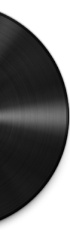
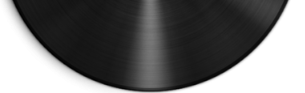
6 days ago
6 days ago
Hey PaperLedge crew, Ernis here, ready to dive into another fascinating research paper! Today, we're tackling something that's super relevant to anyone interested in the future of AI, especially in areas like image and video generation. We're talking about making AI models faster and more efficient using something called sparse attention.
Now, you might be asking, "What exactly is attention in AI?" Think of it like this: when you're reading a sentence, you don't focus equally on every word. Your brain attends more to the important ones. Similarly, in AI, attention mechanisms help the model focus on the most relevant parts of an image or text when making decisions.
The problem is, traditional attention can be incredibly resource-intensive, especially with large images or long texts. It's like comparing every single word to every other word in a novel. That's a lot of comparisons! This leads to what's called O(n^2) complexity, which basically means the computational cost grows exponentially as the input size increases.
That’s where sparse attention comes in. Instead of looking at everything, it strategically focuses on a smaller, more relevant subset. The paper we're looking at today investigates ways to make sparse attention actually faster and more effective. Because, here’s the thing: a lot of previous attempts at sparse attention haven't consistently delivered on their speed promises. They're often too complex, and AI hardware is evolving so quickly that it's hard to keep up.
So, what did the researchers do? First, they introduced something called Generalized Neighborhood Attention (GNA). Think of GNA like different ways of looking at a neighborhood. You could look at your immediate neighbors (like a sliding window), or you could skip a few houses (a strided sliding window), or you could focus on specific blocks within the neighborhood (a blocked attention). GNA is a flexible way to describe these different approaches to focusing on local regions.
Next, they built a simulator to realistically predict how fast these different GNA approaches could potentially be on modern hardware. This simulator is crucial because it takes into account the nitty-gritty details of how AI chips actually work. It helps them understand the upper bound of possible speedups.
But they didn't stop there! They then implemented GNA on top of a super-fast foundation called FMHA, specifically designed for the NVIDIA Blackwell architecture – the latest and greatest in AI chips. The results? Their implementation was able to achieve the theoretically maximum speedup in many cases, reaching an incredible 1.3 petaFLOPs/second using FP16 precision. Imagine a sports car being able to max out its speedometer and actually going the speed that's marked on it!
Here's where it gets really interesting. They plugged their GNA configurations into existing, cutting-edge AI models like Cosmos-7B, HunyuanVideo, and FLUX – all used for generating images and videos. And guess what? They saw end-to-end speedups of 28% to 46% on B200 chips without any fine-tuning! That’s like getting a significant performance boost on your computer just by swapping out a single component, without having to reinstall everything.
"Our implementation can fully realize the maximum speedup theoretically possible in many perfectly block-sparse cases, and achieves an effective utilization of 1.3 petaFLOPs/second in FP16."
The best part? They're open-sourcing their simulator and Blackwell kernels through the NATTEN project. This means anyone can use and build upon their work!
So, why does this research matter? Well, for:
AI Researchers: This provides a practical, high-performance implementation of sparse attention and a valuable simulation tool.
AI Engineers: This offers a way to speed up existing models without extensive retraining.
Anyone Interested in AI: This shows how clever algorithmic improvements combined with optimized hardware can lead to significant performance gains, making AI more accessible and efficient.
This research is about pushing the boundaries of what's possible with AI, making it faster, more efficient, and ultimately, more useful for everyone. It's a great example of how understanding the underlying hardware and designing algorithms that take advantage of it can lead to big breakthroughs.
Here are a few questions this paper brought up for me:
How might these sparse attention techniques impact the development of even larger and more complex AI models in the future?
What are the potential limitations of GNA, and what other types of sparse attention mechanisms might be worth exploring?
Could these speedups translate to lower energy consumption, making AI more sustainable?
That's all for today's deep dive, PaperLedge crew! I'm really interested to hear what you think about this paper. Let me know your thoughts and questions in the comments. Until next time, keep learning!Credit to Paper authors: Ali Hassani, Fengzhe Zhou, Aditya Kane, Jiannan Huang, Chieh-Yun Chen, Min Shi, Steven Walton, Markus Hoehnerbach, Vijay Thakkar, Michael Isaev, Qinsheng Zhang, Bing Xu, Haicheng Wu, Wen-mei Hwu, Ming-Yu Liu, Humphrey Shi
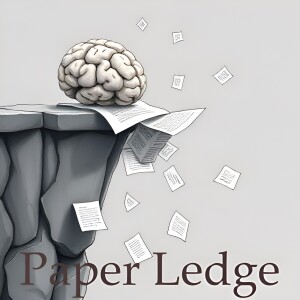
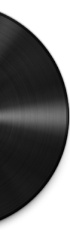
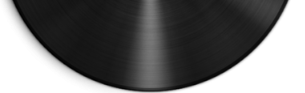
6 days ago
6 days ago
Hey PaperLedge crew, Ernis here! Today, we're diving into a fascinating paper about teaching robots to learn by watching, but with a cool twist that makes the process way more efficient. Think of it like this: imagine you're trying to learn how to bake a cake. The traditional way is to have a master baker show you exactly what to do, step-by-step, using only perfect, expert demonstrations. That's like current imitation learning methods – they need tons of perfect examples to get things right.
But what if you could learn even from watching someone who messes up a little? Or even just watches the master baker without actually doing anything themselves? That's the problem this paper tackles. The researchers have developed a new method called Latent Diffusion Planning (LDP), and it's all about making robots smarter and more adaptable learners.
So, how does LDP work its magic? Well, it's a bit like having a robot brain that's divided into two key parts:
The Planner: This part is like the robot's internal GPS. It figures out the overall plan for achieving a goal, like navigating a maze or stacking blocks. Crucially, it can learn this plan just by observing – even if those observations aren't perfect demonstrations of the task. This is where the action-free demonstrations come in handy! Think of it as the robot watching a video of someone playing a game, and learning the general strategies without needing to control the character itself.
The Action Taker (Inverse Dynamics Model): This part figures out the specific actions the robot needs to take to follow the plan. It’s like the robot’s hands and feet. Now, here's the cool part: this part can learn from data where things didn't go perfectly, like when someone almost dropped a block but managed to catch it. Imperfect data, but still useful!
The secret sauce that makes this work is the "latent space." Think of it as a simplified, compressed version of reality. Instead of the robot having to process every single pixel of every image it sees, it can focus on the most important features – the things that really matter for understanding the scene and planning actions. This makes everything much more efficient.
The researchers train both the planner and the action taker using a "diffusion objective." This means they use a process of gradually adding noise to data and then learning to remove it. It's like teaching the robot to see through the fog and find the underlying pattern.
So, why does this matter? Well, for a few reasons:
For robotics researchers: LDP offers a more efficient and flexible way to train robots, allowing them to learn from a wider range of data sources.
For AI developers: This approach could be applied to other areas of AI, such as self-driving cars or virtual assistants, where learning from imperfect or incomplete data is crucial.
For everyone else: As robots become more integrated into our lives, it's important that they can learn quickly and adapt to new situations. LDP is a step in that direction.
The results of the paper are pretty impressive. The researchers tested LDP on simulated robotic manipulation tasks, like stacking blocks and moving objects, and it outperformed other state-of-the-art imitation learning methods. This is because LDP can leverage all that extra data that other methods can't use.
This research really opens up some interesting questions. For example:
How well does LDP transfer to real-world robots, where the data is even more noisy and unpredictable?
Could we use LDP to teach robots more complex tasks, like cooking or assembling furniture?
What are the ethical implications of training robots to learn from potentially biased or misleading data?
I'm excited to see what the future holds for LDP and other imitation learning techniques. It's a fascinating area of research with the potential to transform the way we interact with robots. Credit to Paper authors: Amber Xie, Oleh Rybkin, Dorsa Sadigh, Chelsea Finn
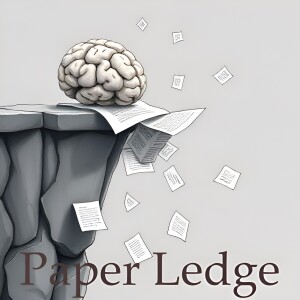
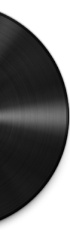
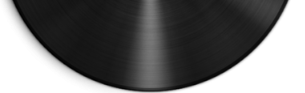
Tuesday Apr 22, 2025
Tuesday Apr 22, 2025
Hey PaperLedge crew, Ernis here, ready to dive into some fascinating research! Today, we're tackling a paper that's all about making AI fairer, and specifically, how to keep those super-smart Large Language Models, or LLMs, from accidentally picking up and spreading biases.
Think of LLMs like really absorbent sponges. They soak up all the information they're trained on – which is a massive amount of text from the internet. But what happens if that text contains biases, maybe stereotypes about certain groups of people? Well, the sponge soaks that up too, and the AI starts reflecting those biases in its responses. Not cool!
So, researchers have been trying to figure out how to "de-bias" these models. One approach is to carefully craft prompts – those questions or instructions you give the AI – to try and steer it away from biased responses. But, the paper we are discussing today points out that this approach is super sensitive. Change the prompt even a little, and the bias can come creeping back.
Another way is to "fine-tune" the model, basically re-training it on a special dataset that's designed to be fair. But that takes a lot of computing power and can also cause the AI to forget other things it learned – kind of like wiping the sponge clean, but accidentally erasing some useful information along with the biases.
"Existing methods are either too sensitive to prompt changes or too computationally expensive and prone to forgetting."
That's where this new paper comes in! It introduces a method called FairSteer. The cool thing about FairSteer is that it doesn't require any special prompts or re-training. It works its magic during the inference stage – that's when the AI is actually generating its responses.
Here's the analogy I like: imagine the AI's brain is a complex network of roads. When it's about to say something biased, it's like a car is about to drive down a road that leads to a biased outcome. FairSteer is like a GPS that subtly nudges the car onto a slightly different road, one that leads to a fairer destination.
How does it work? Well, the researchers discovered that "fairness-related features" – things that contribute to bias – are encoded in specific directions within the AI's "hidden activation space." Think of that activation space as a multi-dimensional map of all the AI's internal thoughts.
First, FairSteer trains a tiny, lightweight program to detect those "bias signatures" in the AI's thoughts.
Second, it figures out the "debiasing steering vector" (DSV). This is like calculating the direction and strength of the nudge needed to steer the AI away from the biased road. They do this by using small, contrasting prompts.
Third, and finally, during the inference stage, FairSteer subtly adjusts the AI's activations using those DSVs, guiding it towards fairer responses.
The researchers tested FairSteer on six different LLMs and found that it worked really well across a range of tasks, including answering questions, evaluating hypothetical situations, and even generating creative text. The best part? It didn't require any prompt engineering or model retraining!
So why does this matter? Well, for developers, it offers a practical way to make their AI systems fairer without huge computational costs. For users, it means interacting with AI that's less likely to perpetuate harmful stereotypes. And for society as a whole, it's a step towards building AI that's more equitable and just.
Consider this:
If AI is increasingly used to make important decisions – like loan applications or even criminal justice – how crucial is it that we ensure these systems are free from bias?
FairSteer seems promising, but how can we ensure that methods like this don't introduce unintended consequences or new forms of bias?
With the code being released, what are the possible beneficial and harmful applications that could arise from this debiasing technique?
This is a really promising step toward making AI fairer for everyone. And the fact that it's efficient and doesn't require re-training is a game-changer! I am excited to see how this research evolves and the impact it has on the field. Until next time, keep learning and stay curious!
Credit to Paper authors: Yichen Li, Zhiting Fan, Ruizhe Chen, Xiaotang Gai, Luqi Gong, Yan Zhang, Zuozhu Liu
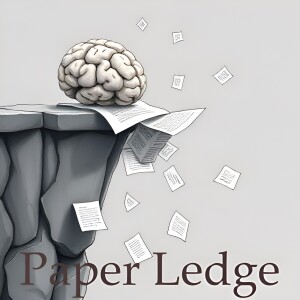
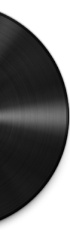
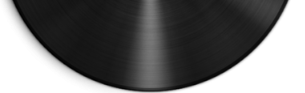
Tuesday Apr 22, 2025
Tuesday Apr 22, 2025
Alright learning crew, Ernis here, and welcome back to PaperLedge! Today, we're diving into a fascinating area of AI: how to make those giant, brainy AI models actually usable without breaking the bank. Think of it like this: imagine you have a super-smart friend who knows everything, but every time you ask them to help with a specific task, you have to rewrite their entire brain! That's essentially what "fine-tuning" large AI models used to be like – incredibly resource-intensive.
This paper we're discussing offers a roadmap to navigate a new approach called Parameter-Efficient Fine-Tuning, or PEFT. Sounds technical, but the core idea is brilliant: instead of rewriting the entire brain (the whole model), PEFT lets us tweak just a small, relevant part. It's like giving your super-smart friend a specific new module or skill without changing their core knowledge.
So, why is this important? Well, large models like those powering chatbots and image recognition are amazing, but they require enormous computing power and data to adapt to specific tasks. This makes them inaccessible to many researchers and smaller companies. The paper highlights these key issues:
Overfitting: Imagine teaching your friend too specifically for one task. They might forget general knowledge!
Catastrophic Forgetting: Similar to above, but even worse – they completely forget old information when learning something new.
Parameter Inefficiency: Rewriting the entire brain every time is just plain wasteful!
PEFT tackles these head-on by only updating a small percentage of the model's parameters. This saves time, energy, and money!
The paper then breaks down PEFT methods into a few main categories. Think of it like different ways to add that new skill module to your super-smart friend:
Additive Methods: These add new, small components to the existing model. Like giving your friend an external hard drive with new information.
Selective Methods: These only train specific parts of the model, leaving the rest untouched. Like focusing on improving your friend's math skills while their language skills remain the same.
Reparameterized Methods: These cleverly change how the model learns, making it more efficient. Like finding a new study technique that helps your friend learn faster.
Hybrid Methods: A mix-and-match of the above!
Unified Frameworks: These aim to bring together the best aspects of all the other methods.
The authors systematically compare these methods, weighing their strengths and weaknesses. They explore how PEFT is being used across various fields, from language processing to computer vision and even generative models. The results are impressive – often achieving performance close to full fine-tuning but with significantly less resource consumption.
But it's not all sunshine and roses! The paper also points out some challenges:
Scalability: Can PEFT work effectively with even larger models?
Interpretability: Can we understand why a PEFT method works?
Robustness: How well does PEFT perform when faced with unexpected or noisy data?
The authors also suggest exciting future directions, like using PEFT in federated learning (training models across multiple devices without sharing data) and domain adaptation (adapting models to new situations). They even call for more theoretical research to understand the fundamental principles behind PEFT.
"Our goal is to provide a unified understanding of PEFT and its growing role in enabling practical, efficient, and sustainable use of large models."
In essence, this paper argues that PEFT is a crucial step towards democratizing AI, making these powerful models accessible to a wider range of users and applications.
So, as we wrap up, let's ponder a few questions. First, do you think PEFT will become the de facto standard for fine-tuning large models? Second, how might PEFT impact industries that currently struggle with the high costs of AI? And finally, could PEFT inadvertently lead to the creation of even more biased or unfair AI systems if not carefully implemented and monitored? Let me know your thoughts in the comments. Until next time, keep learning!Credit to Paper authors: Nusrat Jahan Prottasha, Upama Roy Chowdhury, Shetu Mohanto, Tasfia Nuzhat, Abdullah As Sami, Md Shamol Ali, Md Shohanur Islam Sobuj, Hafijur Raman, Md Kowsher, Ozlem Ozmen Garibay