PaperLedge
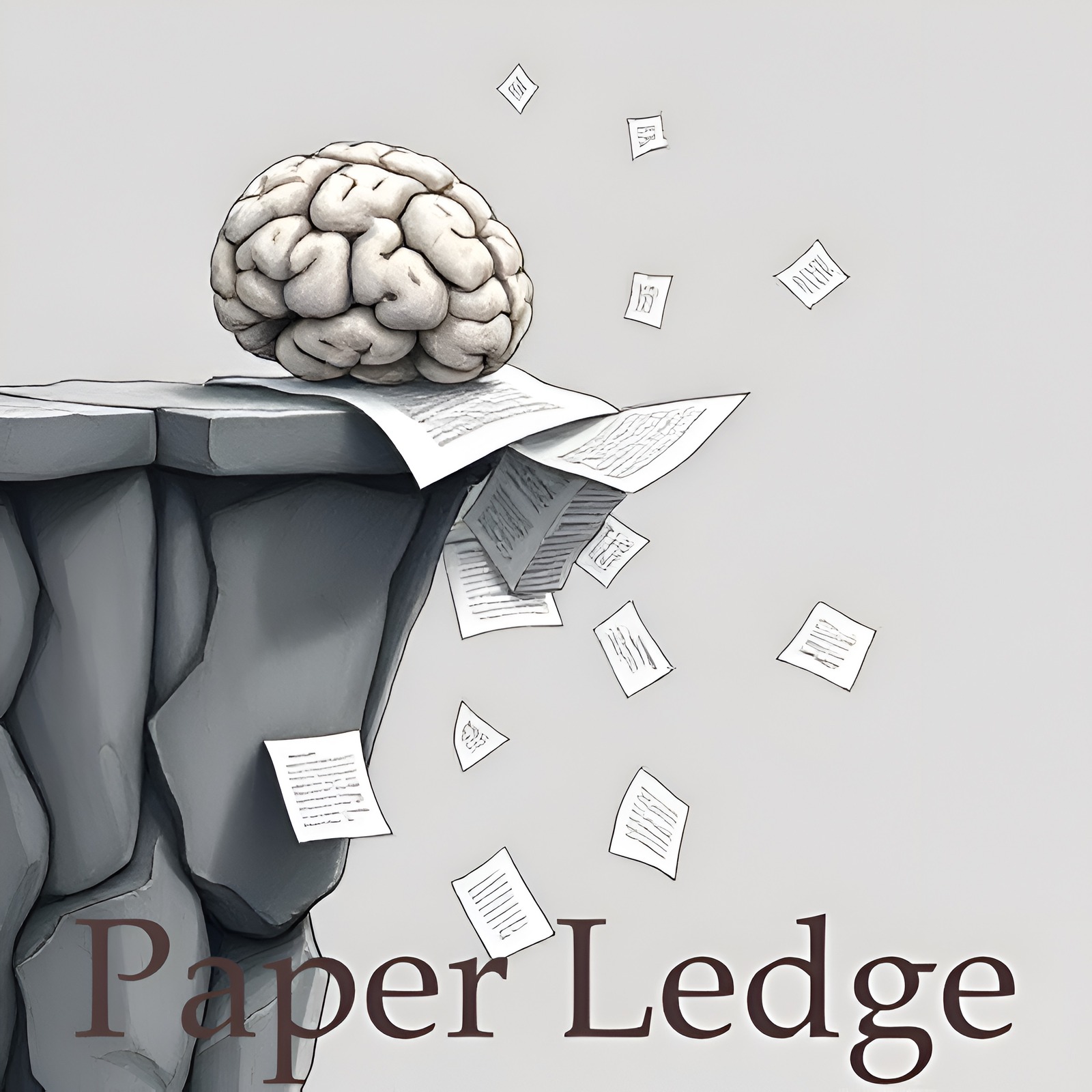
PaperLedge where research meets storytelling is a revolutionary podcast where cutting-edge research meets AI-powered storytelling. Hosted by the Ernis, whose blend of gentle reassurance, cosmic wonder, explanatory clarity, and enthusiastic charm makes complex research accessible to everyone. Each episode, Ernis transforms the latest academic papers into engaging, jargon-free audio experiences that deliver key insights in digestible formats. Whether you’re a researcher seeking interdisciplinary perspectives, a student supplementing your studies, or simply curious about scientific breakthroughs, PaperLedge has something for you.
Episodes
Episodes
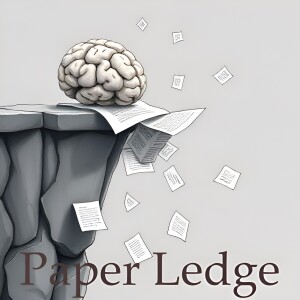
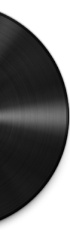
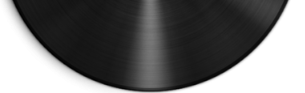
Monday Jun 02, 2025
Monday Jun 02, 2025
Hey learning crew, Ernis here, ready to dive into some seriously cool research! Today we're talking about how computers generate text, like writing stories, code, or even solving math problems. Think of it like this: you give the computer a prompt, and it has to fill in the blanks to create something new.
Now, there are two main ways computers do this. One way, called autoregressive models (ARMs), is like writing a sentence one word at a time, always looking back at what you've already written. It's like building a LEGO tower brick by brick.
But there's a newer, cooler method called masked diffusion models (MDMs). Imagine a Mad Libs game where some words are blanked out, and the computer has to guess what goes in those blanks. That's basically what MDMs do. They've become really good, almost as good as the "brick by brick" method!
But here's the thing: usually, everyone focuses on making the results better, like making the computer's writing more creative or accurate. Nobody really looked at making the process faster...until now!
This paper introduces something called EB-Sampler. Think of it like a turbocharger for MDMs. The researchers realized that when you mask out some words, often, figuring out one masked word actually tells you what several other masked words should be automatically! It's like if you know the first letter of a word in a crossword puzzle, it drastically narrows down the possibilities for other words connected to it.
The EB-Sampler uses this idea to cleverly unmask multiple words at once, without sacrificing accuracy. It's like instead of filling in one blank in the Mad Libs at a time, you strategically fill in a few that give you clues to the rest.
The researchers even developed a whole framework for understanding how this "adaptive unmasking" works and how much error it might introduce. They wanted to make sure they weren't just speeding things up at the cost of making a mess.
And guess what? It works! EB-Sampler makes these MDMs run 2-3 times faster on things like coding and math problems. That's a huge improvement!
But the really cool part is that this method also works on smaller, more intuitive reasoning tasks, like solving mazes or Sudoku puzzles. These are the types of problems that the "brick by brick" autoregressive models often struggle with. So, this research isn't just about making computers write faster; it's about making them think more efficiently.
So, why does this matter?
For coders and developers: Faster code generation means faster software development and more powerful AI tools.
For researchers: This opens up new avenues for exploring how AI models reason and solve problems.
For everyone: More efficient AI means less energy consumption and more accessible technology.
"EB-Sampler accelerates sampling from current state of the art MDMs by roughly 2-3x on standard coding and math reasoning benchmarks without loss in performance."
This research is a reminder that sometimes, the biggest breakthroughs come from looking at problems in a new way, not just by throwing more computing power at them.
Now, a few things that came to mind while reading:
Could this EB-Sampler approach be applied to other types of AI models besides language models?
How does the "error tolerance" in EB-Sampler affect the creativity or originality of the generated text or solutions?
What are the potential limitations of EB-Sampler? Are there certain types of tasks where it might not be as effective?
Food for thought, learning crew! Until next time, keep exploring!Credit to Paper authors: Heli Ben-Hamu, Itai Gat, Daniel Severo, Niklas Nolte, Brian Karrer
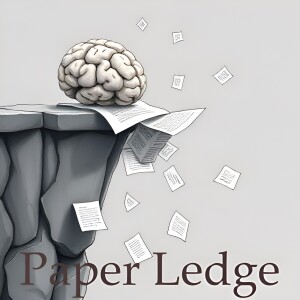
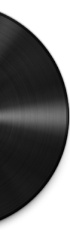
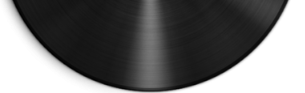
Monday Jun 02, 2025
Monday Jun 02, 2025
Hey PaperLedge crew, Ernis here, ready to dive into some fascinating research that touches on something we all deal with: trusting what we hear, especially from AI.
Today, we're talking about Large Language Models – think of them as super-smart chatbots like ChatGPT or Bard. They can write poems, answer questions, and even generate code. But here's the thing: how much can we really trust them?
This paper tackles a crucial issue: do these AI models accurately communicate how certain they are about the information they’re giving us? Imagine a friend who confidently tells you something, but they're actually just guessing. That's not great, right? It's even worse when it comes from an AI because we might rely on it for important decisions.
The researchers call this "faithful confidence calibration." Basically, it's about making sure that when an LLM is uncertain, it sounds uncertain. It shouldn’t be spitting out answers with complete confidence if it's really just making an educated guess.
Think of it like this: if you ask an LLM about the capital of Uzbekistan, and it's not 100% sure, it should say something like, "I think it's Tashkent, but I'm not completely positive." It shouldn't declare "The capital of Uzbekistan IS Tashkent!" with unwavering certainty if it's pulling information from a potentially unreliable source.
What the researchers found is a bit alarming: LLMs are generally terrible at this! They often sound very confident even when they're wrong. This can lead us to over-rely on them and erode our trust over time. Existing attempts to fix this problem, such as tweaking the prompts or focusing solely on factual accuracy, haven't been very effective. Some even make the problem worse!
But don't despair, crew! The researchers didn't just point out the problem; they also came up with a solution. They developed a new prompting technique called MetaFaith. It's inspired by human metacognition - our ability to think about our own thinking.
MetaFaith essentially encourages the LLM to reflect on how confident it should be before answering. It's like asking the AI to double-check its sources and consider its own limitations before opening its digital mouth.
The results were impressive! MetaFaith significantly improved how faithfully the LLMs communicated their uncertainty. In fact, human judges preferred the MetaFaith-generated responses a whopping 83% of the time! That’s a huge win for honesty and reliability in AI.
So, why does all of this matter?
For developers: It highlights the need to prioritize faithful confidence calibration when building and deploying LLMs.
For everyday users: It reminds us to be critical of the information we get from AI and to be aware of its potential to be overconfident.
For researchers: It opens up new avenues for exploring how to make AI more trustworthy and reliable.
Now, here are a couple of questions that popped into my head while reading this:
If LLMs are so bad at assessing their own confidence, does this mean they're also bad at assessing the reliability of their sources?
Could techniques like MetaFaith be used to improve other aspects of AI trustworthiness, such as reducing bias or increasing transparency?
This paper really underscores the importance of critical thinking, not just for humans, but also for the AI we're increasingly relying on. It's a call to action to make sure these powerful tools are not just intelligent, but also honest and reliable. What do you all think? Let me know your thoughts in the comments below!Credit to Paper authors: Gabrielle Kaili-May Liu, Gal Yona, Avi Caciularu, Idan Szpektor, Tim G. J. Rudner, Arman Cohan
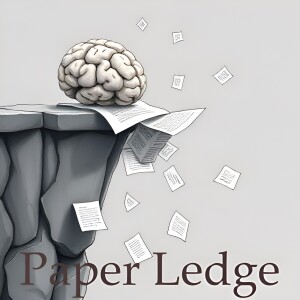
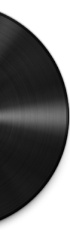
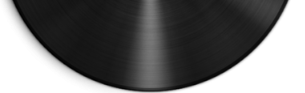
Monday Jun 02, 2025
Monday Jun 02, 2025
Hey PaperLedge crew, Ernis here, ready to dive into some seriously cool AI stuff! Today, we're talking about a new framework that's trying to make AI models not just smarter, but also more efficient in how they think. Think of it like this: imagine you're trying to solve a really tough riddle. Sometimes you need to mull it over, ponder different angles, and let your brain simmer for a bit. Other times, you just get it, and the answer pops right into your head. That's kind of what this research is all about – teaching AI to know when to slow down and think hard, and when to speed up and give us the answer.
The framework is called AlphaOne – or α1 for short – and the researchers are tackling a big problem: Large Reasoning Models, or LRMs. These are AI models designed to handle complex tasks like math problems, writing code, and even tackling scientific questions. The thing is, these models can sometimes be a bit... well, let's just say they can take the scenic route to the answer. They might spend a lot of time "thinking" even when they don't need to.
So, AlphaOne introduces this clever concept called the "α moment." Think of α as a universal knob that controls how much "slow thinking" the AI does. Before the "α moment," the AI's thinking process is like a brainstorming session, but the framework dynamically schedules those slow thinking transitions. After the "α moment," it's like the AI hits a switch and says, "Okay, time to wrap this up and give the answer!"
Here's a relatable example: Imagine you're baking a cake. Before the "α moment," you're gathering ingredients, mixing them slowly, and making sure everything is just right. That's the slow, deliberate part. After the "α moment," you pop it in the oven, and it's all about letting the heat do its work to bake it to perfection!
What's really neat is that AlphaOne uses a fancy mathematical trick – a Bernoulli stochastic process – to decide when to insert those "slow thinking" moments. It sounds complicated, but basically, it's like flipping a coin to decide whether the AI should pause and think a bit more. It's a way to make the thinking process more flexible and efficient. This is something really cool because it allows for a more dense, slow-to-fast reasoning modulation. Think of it as giving the AI the ability to shift gears smoothly, rather than just slamming on the brakes or flooring the gas pedal.
The researchers tested AlphaOne on a bunch of tough problems in math, coding, and science. And guess what? It worked really well! The AI was not only able to solve the problems more accurately, but it also did it more efficiently. It's like getting a better grade on a test while also finishing it faster – a win-win situation!
"AlphaOne unifies and generalizes existing monotonic scaling methods by enabling flexible and dense slow-to-fast reasoning modulation."
Why does this matter?
For students and educators, it means potentially better AI tools for learning and problem-solving. Imagine having an AI tutor that can adapt its teaching style to your individual needs, knowing when to explain things slowly and when to let you figure it out on your own.
For developers and AI researchers, AlphaOne offers a new approach to building more efficient and powerful reasoning models. This could lead to breakthroughs in areas like robotics, natural language processing, and even scientific discovery.
For everyone else, it means AI that is more capable, more reliable, and potentially more accessible. As AI becomes increasingly integrated into our lives, it's important to make sure it's working as effectively as possible.
So, here are a few things that have been swirling around in my head:
Could AlphaOne be adapted to different types of thinking, like creative problem-solving or emotional reasoning?
How might we use this kind of framework to help humans become better thinkers, too? Can we learn from AI's "thinking process" to improve our own cognitive abilities?
What ethical considerations should we keep in mind as we develop AI models that can reason and solve problems at this level?
I hope you found this as fascinating as I did! This stuff is important because it is helping bridge the gap between science and the future. Until next time, keep learning!Credit to Paper authors: Junyu Zhang, Runpei Dong, Han Wang, Xuying Ning, Haoran Geng, Peihao Li, Xialin He, Yutong Bai, Jitendra Malik, Saurabh Gupta, Huan Zhang
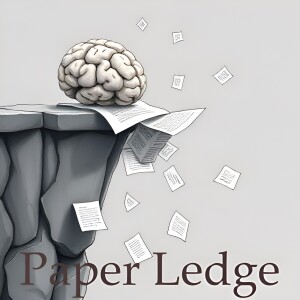
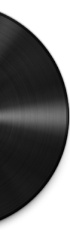
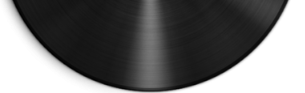
Monday Jun 02, 2025
Monday Jun 02, 2025
Alright Learning Crew, Ernis here, ready to dive into some fascinating research! Today, we're tackling a paper that asks a really important question about AI: Can reinforcement learning actually make language models smarter, or is it just polishing what's already there?
Think of it like this: imagine you're teaching a dog a new trick. You can either reward the dog for almost doing the trick, hoping they eventually figure it out (that's kind of like traditional training). Or, you can use reinforcement learning – rewarding them specifically for each tiny step in the right direction, guiding them towards a completely new behavior they never would have discovered on their own.
This paper looks at whether reinforcement learning (RL) with language models is more like that second scenario. Is it really unlocking new reasoning abilities, or just making the model better at spitting out answers it already knew were likely to get a reward?
The researchers behind this paper argue that, contrary to some popular beliefs, RL can indeed unlock novel reasoning strategies in language models that the original model just couldn't access, no matter how many times it tried! They're calling their approach "ProRL," or Prolonged RL.
Now, what exactly is ProRL? Essentially, they've come up with a special training recipe. It's got a few key ingredients:
KL Divergence Control: Think of this as a gentle nudge to keep the model from straying too far from its original knowledge base while it's learning new things. It's like a safety net!
Reference Policy Resetting: Periodically, they kind of "reset" the model's learning progress, allowing it to explore different paths and avoid getting stuck in a rut.
A Diverse Suite of Tasks: They threw a whole bunch of different challenges at the model to make sure it wasn't just getting good at one specific type of problem.
So, what did they find? Well, the models trained with ProRL consistently outperformed the original models across a wide range of tests. And here's the kicker: even when the original model was given tons of chances to answer correctly, it still couldn't match the performance of the RL-trained model. This suggests that RL isn't just amplifying existing abilities, it's creating new ones.
"Our empirical analysis reveals that RL-trained models consistently outperform base models across a wide range of pass@k evaluations, including scenarios where base models fail entirely regardless of the number of attempts."
Think of it like this: imagine you're trying to solve a complex puzzle. The original model might be able to try a bunch of different combinations of pieces, but it's limited by its initial understanding of the puzzle. ProRL, on the other hand, helps the model develop a new strategy for approaching the puzzle altogether, unlocking solutions it never would have found otherwise.
The researchers also found that the longer they trained the model with ProRL, and the better the original model was at the task, the more its reasoning abilities improved. This suggests that RL can explore and populate new regions of solution space over time.
Why does this matter? Well, for those interested in AI development, it suggests that RL is a powerful tool for building truly intelligent systems. For those concerned about AI safety, it highlights the importance of understanding how RL can shape the reasoning abilities of these models. And for everyone, it raises the exciting possibility of AI that can solve problems in ways we haven't even imagined yet!
Now, this research definitely got my gears turning. Here are a couple of questions that jumped to mind:
Could ProRL be used to teach AI models to think more creatively or ethically?
What are the potential risks of unlocking new reasoning abilities in AI, and how can we mitigate them?
The researchers have even released their model weights, which is awesome! You can find them here: https://huggingface.co/nvidia/Nemotron-Research-Reasoning-Qwen-1.5B
That's all for today's deep dive, Learning Crew! I hope this sparked some curiosity and helped make this research a little more accessible. Until next time, keep learning and keep questioning!Credit to Paper authors: Mingjie Liu, Shizhe Diao, Ximing Lu, Jian Hu, Xin Dong, Yejin Choi, Jan Kautz, Yi Dong
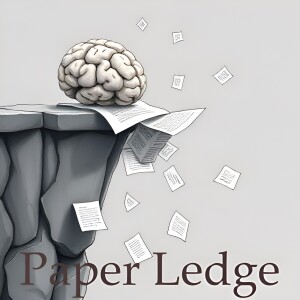
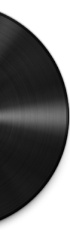
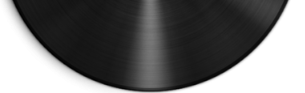
Monday Jun 02, 2025
Computer Vision - SiLVR A Simple Language-based Video Reasoning Framework
Monday Jun 02, 2025
Monday Jun 02, 2025
Hey PaperLedge crew, Ernis here, ready to dive into some seriously cool AI research! Today, we're talking about making AI see and understand videos like never before. Think of it as leveling up AI's ability to watch and really get what's happening, not just seeing moving pictures.
So, you know how those super-smart Large Language Models, or LLMs, are acing math problems and writing code? They're like the star students in the AI world. But when it comes to videos, especially complex ones that need real understanding, they kind of…struggle. It's like they can see the pieces but can't quite put the whole puzzle together, especially when audio and speech are involved.
That's where the researchers behind this paper stepped in. They came up with a system called SiLVR - and it stands for "Simple Language-based Video Reasoning". It's a clever way to help AI break down and understand videos.
Think of it like this: Imagine you're trying to explain a complicated movie scene to someone who hasn't seen it. You wouldn't just show them the raw footage, right? You'd probably describe the key moments, maybe point out important dialogue, and summarize what's happening. SiLVR does something similar for AI.
It works in two main steps:
Step 1: Language Transformation: Instead of feeding the raw video directly to the AI, SiLVR first turns it into a language-based description. This includes things like short captions for clips, subtitles from speech, and even information extracted from the audio itself. It's like creating a detailed written summary of the video.
Step 2: Reasoning with Language: Then, it feeds that language description to a powerful LLM. The LLM can now use its language skills to reason about what's happening in the video. It can answer questions, make predictions, and generally understand the video at a much deeper level.
Now, here's where it gets really interesting. Videos can be long, and all those language descriptions can add up to a lot of information. To handle this, SiLVR uses what they call an "adaptive token reduction scheme." Think of it like this: if you're watching a long movie, you don't need to pay attention to every single frame. You can skip over the boring parts and focus on the key scenes.
The adaptive token reduction scheme works similarly. It dynamically figures out which parts of the language description are most important and focuses on those, saving processing power and improving efficiency. It's like having a smart editor who knows exactly what to cut to keep the story moving.
The results are impressive! SiLVR achieved the best-reported results on a bunch of benchmarks designed to test video understanding. This means it's better at understanding complex videos than other AI systems, especially on tasks that require reasoning about long-term events, cause and effect, and knowledge acquisition.
Here's a quote that really stood out to me from the paper:
"...strong reasoning LLMs can effectively aggregate multisensory input information from video, speech, and audio for complex temporal, causal, long-context, and knowledge acquisition reasoning tasks in video."
In simpler terms, even though these LLMs weren't specifically trained on videos, they can still use the language descriptions created by SiLVR to understand what's going on, drawing information from the video, speech, and audio.
Why does this matter? Well, think about it. Better video understanding could lead to:
More accurate video search and recommendation systems
Improved AI assistants that can understand and respond to video content
More effective video surveillance and security systems
Even advancements in fields like education and healthcare, where video analysis is crucial. Imagine AI helping doctors analyze medical videos or assisting students with complex video tutorials.
So, as we wrap up, a couple of questions I'm pondering after reading this:
How far can this language-based approach be pushed? Will we eventually reach a point where AI can understand videos as well as humans, even without seeing the raw footage?
Could SiLVR be adapted to other types of multimedia content, like virtual reality or augmented reality experiences?
This research is super promising, and it's exciting to see how AI is learning to see and understand the world around us.
That's all for this week's deep dive. Until next time, keep exploring!Credit to Paper authors: Ce Zhang, Yan-Bo Lin, Ziyang Wang, Mohit Bansal, Gedas Bertasius
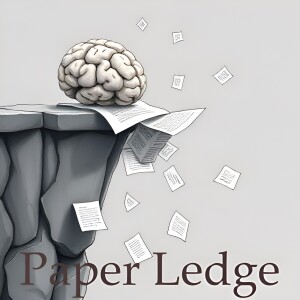
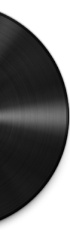
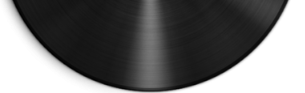
Monday Jun 02, 2025
Monday Jun 02, 2025
Alright learning crew, Ernis here, ready to dive into some cutting-edge AI research! Today, we're talking about making those amazing Multimodal Large Language Models (MLLMs) – you know, the ones that can understand both text and images – even smarter and more reliable.
Think of it like this: you're teaching a kid to bake a cake (the MLLM). You want them to understand the recipe (text) and also recognize when the batter looks right (images). But how do you make sure they really learn and don't just memorize?
That's where Reinforcement Learning with Verifiable Rewards (RLVR) comes in. It's a fancy name, but the core idea is simple: instead of just telling the AI if it's right or wrong, you give it a reward based on whether it can prove its answer. Like, showing its work in math class!
"RLVR is like giving the AI a checklist to make sure it followed all the steps correctly, rather than just saying 'yes' or 'no'."
Now, applying RLVR to these image-and-text models is tricky. It's not just about one task anymore. We're dealing with all sorts of things – recognizing objects, understanding spatial relationships, and using logic. It's like asking that same kid to bake a cake, build a Lego castle, and write a poem – all at the same time!
This particular paper tackled a big problem: How do you train an MLLM using RLVR when you have lots of different datasets, each with its own goals and rewards? Imagine you have a dataset that focuses on identifying objects in images, and another that focuses on answering questions about those images. Training on both at once might confuse the AI. It's like feeding that kid cake and broccoli at the same time – conflicting signals!
So, what did these researchers do? Well, they created a system to intelligently mix these datasets. It's like having a chef who knows exactly how much of each ingredient to use to create the perfect dish. They didn't just throw everything in at random!
Here's the breakdown:
They built a framework to train MLLMs with RLVR on multiple datasets, each with its own "verifiable reward."
They developed a strategy to predict how well the AI would learn based on the mix of datasets. Think of it as a recipe prediction tool!
The result? By carefully mixing the datasets, they significantly improved the MLLM's ability to reason and generalize. In fact, the best mixture improved the model's accuracy by an average of 5.24% compared to just using a random mix of data. And a whopping 20.74% improvement over the original, untrained model!
Why is this important? Well, it means we're one step closer to AI that can truly understand the world around us, not just memorize facts. This could have huge implications for things like:
Robotics: Helping robots understand complex environments and tasks.
Medical imaging: Assisting doctors in diagnosing diseases by analyzing images and text reports.
Accessibility: Creating tools that can describe images for visually impaired people.
This research shows that by carefully designing how we train AI, we can unlock incredible potential.
So, some questions that pop into my head:
Could this data mixing strategy be applied to other types of AI models, not just MLLMs?
How can we make these "verifiable rewards" even more robust and less susceptible to being gamed by the AI?
What are the ethical considerations of using AI trained in this way, especially in sensitive areas like medical diagnosis?
That's all for today's PaperLedge deep dive. Keep learning, keep questioning, and I'll catch you next time!Credit to Paper authors: Yiqing Liang, Jielin Qiu, Wenhao Ding, Zuxin Liu, James Tompkin, Mengdi Xu, Mengzhou Xia, Zhengzhong Tu, Laixi Shi, Jiacheng Zhu
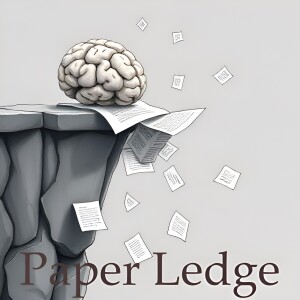
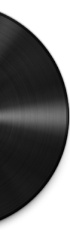
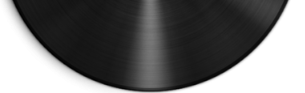
Monday Jun 02, 2025
Monday Jun 02, 2025
Alright learning crew, welcome back to PaperLedge! Today, we're diving into some seriously cool AI research that could change how we interact with those powerful vision-language models, you know, the ones that can "see" and "talk" to us. This paper introduces something called ProxyThinker, and trust me, it's a game-changer.
Think of it this way: imagine you're trying to learn a really complex skill, like playing chess at a grandmaster level. You could spend years training, right? That’s kind of like how these big AI models, called LVLMs, learn visual reasoning. They need tons of data and a whole lot of computational power, especially when using a technique called Reinforcement Fine-Tuning, or RFT.
RFT is like having a really strict coach who constantly gives the AI feedback, pushing it to improve its visual reasoning. But here’s the rub: this “coaching” process is incredibly expensive in terms of computer power. It takes a massive amount of time and energy to train these models using RFT.
That's where ProxyThinker comes in. The researchers behind this paper figured out a clever shortcut. Instead of fully training a giant model with RFT, they found a way for smaller, more specialized “reasoners” to lend their expertise to the big models without any training of the big model itself! It's like borrowing your super-smart friend's brain for a test, but without them actually having to study for you.
How does it work? It's a bit like this: imagine you have a regular painter (the big model) and a master artist (the small, RFT-trained reasoner). The regular painter is good, but the master artist has that extra something, that nuanced understanding. ProxyThinker, in essence, subtracts the regular painter's style from the master artist's style. This difference, this delta, is then subtly applied to the regular painter, allowing them to create a painting that looks much more like the master's work.
Essentially, ProxyThinker modifies how the big model decodes information, making it "think" more like the smaller, smarter reasoner. This allows the large model to demonstrate more sophisticated behaviors, like double-checking its own work or even correcting itself if it makes a mistake!
The results are pretty impressive. ProxyThinker significantly improved the performance of these big models on tricky visual tasks, like spatial reasoning (understanding where things are in relation to each other), mathematical reasoning (solving problems based on what they see), and even multi-disciplinary reasoning (combining knowledge from different areas).
And here's the kicker: ProxyThinker is fast. The researchers implemented it in a way that allows multiple language models to work together in parallel, making the whole process way more efficient. They claim it's up to 38 times faster than other similar methods!
So, why does this matter? Well, for starters, it makes these powerful AI models more accessible. If we don't need to spend a fortune training them, more people can use them. This could be huge for:
Researchers: They can explore new AI capabilities without breaking the bank.
Developers: They can integrate advanced visual reasoning into their applications more easily.
Everyone: Imagine AI assistants that can truly understand the world around them, helping us with everything from navigating unfamiliar places to solving complex problems.
Here are a couple of things that come to mind as I'm digesting this paper:
If ProxyThinker can make big models "borrow" reasoning skills from smaller ones, could we use a similar approach to transfer other kinds of knowledge or abilities?
Could this technique potentially amplify biases present in the smaller, RFT-trained models? And how could we mitigate that?
This is exciting stuff, learning crew! It’s pushing the boundaries of what's possible with AI, and it's doing so in a way that's more efficient and accessible. You can find the code for ProxyThinker over at the GitHub link in the show notes. Go check it out, and let me know what you think!Credit to Paper authors: Zilin Xiao, Jaywon Koo, Siru Ouyang, Jefferson Hernandez, Yu Meng, Vicente Ordonez
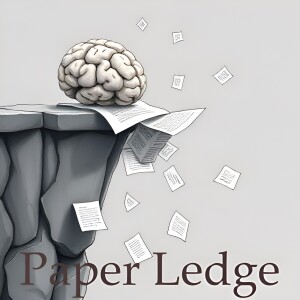
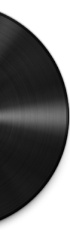
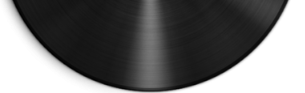
Monday Jun 02, 2025
Monday Jun 02, 2025
Alright learning crew, Ernis here, ready to dive into another fascinating paper! Today, we're tackling a really cool idea that combines the power of brains and logic – think of it as blending the creativity of an artist with the precision of an engineer.
This paper is all about neuro-symbolic learning. Now, that sounds like a mouthful, right? But let's break it down. Imagine you're teaching a computer to play chess. One way is to show it tons of games and let it figure things out using something called a neural network – that's the "neuro" part, inspired by how our brains work. The other way is to give it a set of rules – like "the queen can move any number of squares diagonally or in a straight line" – that's the "symbolic" part, based on logic and reasoning.
Traditionally, neuro-symbolic learning tried to do both at the same time: train a neural network alongside a set of rules. The idea was to get the best of both worlds: the neural network's ability to learn from data and the symbolic rules' ability to provide clear, understandable reasoning. But it turned out to be tricky – like trying to teach a dog new tricks while also forcing it to follow a rigid instruction manual. It often only worked for very simple problems.
Now, fast forward to today, and we have these amazing things called foundation models. Think of them as super-smart computers that have been trained on massive amounts of data – basically, the entire internet! These models are so good that you can just ask them to do things, instead of having to train them from scratch. It's like having a research assistant that already knows a ton about the topic. This is called prompting.
However, even these super-smart models can be a bit… unreliable. They might give you the right answer most of the time, but sometimes they can just make stuff up! Plus, it's hard to know why they gave you a particular answer – it's like a black box.
“Supplementing foundation models with symbolic programs, which we call neuro-symbolic prompting, provides a way to use these models for complex reasoning tasks.”
That's where the "neuro-symbolic" idea comes back in! This paper proposes something called neuro-symbolic prompting. The idea is to use these powerful foundation models, but guide them with symbolic rules. It's like giving your super-smart research assistant a detailed outline and a checklist to make sure they stay on track and their reasoning is sound.
The paper argues that foundation models change the game. Before, you had to train your neural networks from scratch, which took a lot of time, data, and computing power. This led to problems where the models wouldn't work well on new, unseen situations. The paper calls these pitfalls of the traditional approach. For example:
Compute: Training models from scratch requires tons of computing resources.
Data: You need massive datasets to train these models, and getting that data can be difficult and expensive.
Programs: Creating the symbolic rules that work well with neural networks can be very challenging.
By using foundation models and guiding them with symbolic rules, we can overcome these challenges. It's like using a pre-built engine for your car instead of trying to build one from scratch – it's faster, cheaper, and more likely to work well!
So, why does this all matter? Well, imagine you're building a self-driving car. You want it to be able to navigate roads safely and reliably. By combining the power of foundation models with symbolic rules, you can create a system that's both intelligent and trustworthy.
Or, imagine you're a doctor trying to diagnose a patient. You can use a foundation model to analyze their medical history and symptoms, but you can also use symbolic rules to ensure that the diagnosis is based on sound medical principles.
This approach has the potential to make AI systems more:
Reliable: Less likely to make mistakes.
Interpretable: Easier to understand why they made a particular decision.
Generalizable: Able to work well in new and unseen situations.
In essence, this paper suggests that foundation models offer a fresh start for neuro-symbolic learning, allowing us to achieve its original goals without getting bogged down in the complexities of training from the ground up.
Now, a couple of things to chew on after digesting all that:
If foundation models are so powerful, how do we ensure the symbolic rules we use to guide them aren't biased or flawed, leading to unintended consequences?
How do we best determine the right balance between relying on the "brain" (neural network) versus the "logic" (symbolic rules) in different situations? Is there a one-size-fits-all approach, or does it depend on the specific problem?
That’s all for this episode, learning crew. Until next time, keep those neurons firing, and don’t forget to apply a little logic along the way!Credit to Paper authors: Adam Stein, Aaditya Naik, Neelay Velingker, Mayur Naik, Eric Wong